The role of digital and integrative pathology for the detection of translocations: a narrative review
Introduction
Digital pathology consists of different technologies capable of transforming the “classic” workflow into a fully automated and tracked path (1) that culminates with the employment of whole slide imaging (WSI) for primary diagnosis, consultation or multidisciplinary discussions (2-4). Although it can represent an invaluable source of information and a long-term investment with high returns, the diffusion of digital pathology is still slowly progressing, leading some authors to postulate possible reasons for this reluctance (5). To address these perplexities and provide a handy and step-by-step guidance for this transition, some international societies released detailed recommendations and guidelines for the progressive implementation of digital pathology (6,7). These could represent the basis for the deployment of a further, “third revolution” in pathology (8) represented by the application of artificial intelligence (AI) tools for both the clinical and research activity (9). In line with the necessary integration among different subspecialities for the final comprehensive characterization of cancer (e.g., radiology, molecular biology, and immuno-oncology) (10), digital pathology can play a new emerging pivotal role. Thus, the present review will focus on the advancements of digital and computational pathology in prediction oncology for the detection and prevision of translocations, which can be relevant as specific targets for candidating patients to molecular therapies. We present the following article in accordance with the Narrative Review reporting checklist (available at https://pcm.amegroups.com/article/view/10.21037/pcm-21-56/rc).
Methods
A systematic literature search was conducted on different research engines (PubMed, IEEE Xplore, dblp, ACM digital library, Inspec) using the terms: “digital pathology AND translocations”; “digital pathology AND mutations”; “artificial intelligence AND translocations”; “deep learning AND translocations”; “deep learning AND mutations”; “digital pathology AND lung cancer”, over a 15-year interval from January 1, 2006 to October 31, 2021 (Table 1). The research led to 2,216 results, from which 118 duplicates and 11 articles in non-English language were removed (Figure 1). For the obtained 2087 articles, abstracts were evaluated by two of the authors (CB and SC) and screened for manuscripts focused on the detection of mutations/translocations from digital pathology tools. Discordances on the selection of manuscripts have been resolved through the re-evaluation by a third author (VL or FP). For these articles (n=17) the full manuscript has been revised and from the references additional articles have been added to the present review.
Table 1
Items | Specification |
---|---|
Date of search (specified to date, month, and year) | November 15, 2021 |
Databases and other sources searched | PubMed, IEEE Xplore, dblp, ACM digital library, Embase, Inspec. |
Search terms used | “Digital pathology AND translocations”; “digital pathology AND mutations”; “artificial intelligence AND translocations”; “deep learning AND translocations”; “deep learning AND mutations”; “digital pathology AND lung cancer” |
Timeframe | January 1, 2006 to October 31, 2021 |
Inclusion and exclusion criteria (study type, language restrictions, etc.) | From the original literature search have been removed: duplicates, non-English articles, review/non-original data, paper based on unclear methods, manuscripts focused on the assessment of mutation/translocation applying digital tools on other sources (no WSI). |
Selection process (who conducted the selection, whether it was conducted independently, how consensus was obtained, etc.) | Two of the authors (CB and SC) conducted the literature research, independently evaluating the abstracts and screening for manuscripts focused on the detection of mutations/translocations from digital pathology tools. Discordances on the selection of manuscripts have been resolved through the re-evaluation by a third author (VL or FP). |
WSI, whole slide imaging.

Digital pathology workflow: essential requirement for AI implementation
The implementation of WSI as a surrogate of the glass slides is just one of the steps required for the transition towards a digital pathology workflow (11). Actually, this represents the last stage of the progressive modifications that should affect the anatomic pathology laboratory, starting from the accessioning to the reporting and archiving phases, as stressed by the most recent recommendations (7). One of the most important advancements in this direction is represented by the introduction of a completely tracked and fully automated laboratory workflow, aided by the implementation of barcodes to uniquely identify each case/patient along the diagnostic process. This is further enhanced by the employment of dedicated barcode readers and cameras at grossing, embedding, and sectioning stations (1), helping to significantly reduce the rate of errors due to missing/under-represented material on the WSI. This is further facilitated by the complete integration with the laboratory information system (LIS), an essential interface that plays different roles in all phases of patient testing, including specimen and test order entry, specimen processing and tracking. Moreover, the adoption of a LIS-centric approach would allow an easy integration of the WSI in the diagnostic routine of every pathologist independently from the vendors of scanners and supplies used in the laboratory. These are the main and basic requirements to lay the foundations for the adoption of AI tools for the detection of mutations/translocations in human cancer.
Adding a molecular dimension to digital pathology: the detection of mutations in cancer
The application of AI tools coupled with digital pathology already demonstrated a complementary role in traditional histology diagnoses (12-14). Moreover, a prognostic application was evaluated, predicting a metastatic aggressive behavior from hematoxylin and eosin (H&E) stained sections of the primary tumor (15). The most fascinating aspect is related to the possibility of predicting specific cancer-related mutations from phenotypic data. This is not surprising since the neoplastic cell phenotype (nuclear and cytoplasmic texture, size, shape) is, after all, conferred by genetic alterations, so individual gene mutations can directly be predicted by AI from H&E slides observing morphological patterns. It should be emphasized that imaging techniques can only identify genetic variants whom directly imprinted tissue morphology; AI algorithms cannot be applied in cases where actual variant allele frequencies of selected mutations impact the classification and prognosis of individual nosological entities, as some hematologic neoplasms (16). With a convolutional neural network (CNN) trained with WSIs of H&E stained hepatocellular carcinoma (HCC), Chen et al. demonstrated how four of the ten most common prognostic and mutated genes in HCC (CTNNB1, FMN2, TP53, and ZFX4) were correctly identified by AI (17). Their method demonstrated high performances at recognizing tumors from normal liver tissue (AUC =0.961; 95% CI: 0.939–0.981) in the validation set. Moreover, testing the mutation prediction capabilities of the model on the region/tiles-level, five of the ten most significant gene mutations, including ARID1A (P=0.036), CTNNB1 (P<0.0001), FMN2 (P=0.0003), TP53 (P=0.0011) and ZFX4 (P=0.0054), showed significant differences between mutated and wild type groups, with the AUCs from 0.71 to 0.89 in the external validation set. Tsou et al. trained a CNN to predict the most common oncogenic drivers in papillary thyroid carcinoma (PTC), BRAF V600E and RAS, based selected ROI on H&E slides. Their work showed good results and, interestingly, the model performed better in predicting RAS than BRAF mutations with a rate of successful prediction from 63.6% and 90% for the BRAF group and RAS group, respectively (18). Indeed, future potential applications of this approach could lead to a reduction of the turnaround times that are generally delayed by the need of genetic testing and to implement a reflex-test approach, limiting the molecular confirmation only to those cases highlighted by the algorithm. The possibility of acting in a completely agnostic fashion, by approaching the molecular assessment of the disease irrespective of the cancer site of origin could be the final goal. For microsatellite instability (MSI) status, deep learning (DL) has been used in different malignancies, distinguishing features predictive of MSI in gastric and colorectal cancer samples (19), even demonstrating high performance at predicting MSI on H&E-stained WSIs (AUC of 0.865 vs. 0.605), with ground truth microsatellite status determined using mismatch repair protein immunohistochemistry (IHC) and MSI-PCR (20).
Adding a molecular dimension to digital pathology: a possible role in the detection of translocations
One of the first fields in which the detection of translocations has been investigated with digital/integrative pathology tools is probably represented by hemato-oncology, in which different entities are characterized by specific and sometimes pathognomonic chromosome rearrangements. Acute promyelocytic leukemia (APL) carries in 95% of cases a balanced chromosomal translocation between chromosomes 15 and 17 t(15;17)(q24; q21) resulting in the formation of the PML-RARA fusion protein (21,22). Molecular techniques require time and are not available in many countries with limited healthcare resources. In contrast, the peripheral smear is universally available, and easily and rapidly obtained in all healthcare settings. For these reasons, some authors investigated whether DL approaches could find morphological features predictive of the molecular translocation status for a correct identification of APL from other subtypes of myeloid leukemias from cellular morphology (23). The proposed model on a discovery cohort of 82 patients and tested on an independent prospective validation cohort of 24 patients had a ROC AUC of 0.822 in the discovery cohort and 0.739 in the validation cohort with analysis based only on the assessment of immature myeloid cells, with lower performances when considering the whole cellular sample. Interestingly, for the phenotypic classification the AI focused on cytoplasmic pixels in non-APL leukemias and nuclear pixels in APL, suggesting some differences in the dispersion of chromatin on Wright staining which could be more focused at the edge of non-APL leukemias cells and more condensed at the center of the APL cells. In Diffuse Large B-cell Lymphomas (DLBCL), the evidence of rearrangement of MYC oncogene demonstrated a poor prognostic value, defining a subset of high-grade lymphomas when in association with rearrangements of Bcl2/Bcl6 (24). Although MYC IHC has been proposed as a surrogate marker, a reliable cut-off to reduce the interobserver reproducibility and to improve the performances of this marker is still missing (25). For this reason, Swiderska-Chadaj et al. developed a DL model to detect MYC rearrangement in WSI from DLBCL on a first multi-center data cohort of 91 cases and a test set of 66 WSIs (26). This approach led to an accuracy of 0.77, with 0.88 and 0.66 of sensitivity and specificity, respectively. They further validated their findings on a larger cohort (245 patients) additionally using CD20 IHC to define the tumor area, with a 0.90/0.95 and 0.52/0.53 of sensitivity and specificity for the internal and external validation sets, respectively (27). The algorithm gives a fast and cheap pre-screening that can direct the application of genetic tests for MYC rearrangement as compared to MYC IHC that shows a lower sensitivity (0.86 for IHC versus 0.93 for the algorithm) and a lower specificity (0.36 for IHC vs. 0.52 for the algorithm) (28). One of the most interesting and stimulating aspects of this approach would be the investigation of the “false negative” results with MYC IHC, which could be compensated by the higher sensitivity of the AI model allowing it to detect a further segment of patients with MYC rearrangements. The introduction of AI tools for the detection of translocation has not been restricted to the hemato-oncology field, with some solid tumors already experiencing the benefits of such digital/integrative pathology techniques. Some specific subtypes of renal cell carcinoma (RCC), in example, are characterized by specific chromosomal translocations (e.g., Xp11.2 leading to the fusion transcript involving TFE3 protein), which can show indistinguishable morphological features from the most common clear cell histotypes (29,30). In this setting, Cheng et al. applied a machine learning model to identify Xp11.2 translocation based on WSI comparing TFE3-RCC and clear cell RCC (ccRCC) (31). Starting from two different dataset of H&E stained WSIs (148 images in total) with a 1:1 TFE3-RCC—ccRCC ratio (TFE3-RCC diagnosis confirmed by FISH), they found an AUC ranging from 0.842 to 0.894, stressing the ability of this model to capture subtle morphological differences between TFE3-RCC and ccRCC. Finally, the application of such digital pathology tools can even facilitate the assessment of routine tests (e.g., fluorescence in situ hybridization FISH) for the detection of some chromosomal abnormalities, as in the case of breast cancer, in which HER2 gene amplification is found in almost 20% of invasive forms and patients with this alteration could benefit from targeted therapy (32). Prediction of HER2 rearrangement is determined by assessing HER2 status with IHC and reflex testing of doubtful IHC (2+) with ISH. Although some oncology societies stressed the need of standardization through automation of the FISH procedure (for HER2 as well as for ALK, ROS1 and NTRK) (33), these slides are still mainly analysed manually by an epifluorescent microscope. Some authors already tried to validate the standardized detection of HER2 gene status using a fully automated ISH procedure, obtaining an overall agreement after revision of 99.4% (κ=0.97) between Leica FISH (automatic) and Abbott FISH on a cohort of 328 invasive breast cancers (34). Moreover, the application of further AI algorithms on FISH digitized slides can provide good picture quality, reducing the analysis time and allowing easy picture archiving and remote diagnostics compared to manual FISH, making HER2 FISH evaluation more efficient (35).
Adding a molecular dimension to digital pathology: focus on lung cancer and its translocations
Over the last 20 years, significant insights into the heterogeneous nature of non-small cell lung cancer (NSCLC), particularly adenocarcinoma, have motivated new research areas endeavoring to elucidate additional actionable characteristics of tumor cells and the surrounding tumor microenvironment. In the era of precision medicine, the identification of targetable somatic mutations is an essential step in determining optimal systemic therapy in patients with adenocarcinoma of pulmonary origin. During these years, different efforts have been made to decipher subtle morphological differences that can underpin specific genetic alterations of NSCLC (36,37). In this setting, the application of innovative AI techniques represent a promising source of information that can complement the “classic” histopathology, as already demonstrated by the capability of these tools to correctly detect (38) and classify NSCLC subtypes (39), predicting its prognosis (40) and the presence of metastatic lymph nodes (41). Recently, Coudray et al. using a deep CNN correctly classified lung tumors as adenocarcinoma and squamous cell carcinoma, with an AUC of 0.97 (vs. human eye), and predicted 6 out of 10 mutations (STK11, EGFR, FAT1, SETBP1, KRAS, and TP53) by the analysis of WSIs with AUCs from 0.733 to 0.856 (42). This can be strategically applied in the future in delicate cases with scant material insufficient to perform additional mutational analysis in frail patients that cannot undergo further bioptic procedures as a possible alternative to the liquid biopsy. Although in the last decade different automated systems have been proposed to scan and score FISH samples for the assessment of ALK (43), ROS1, RET and NTRK aberrations (44), leading to a change in the paradigm of the optimal workflow for digitized FISH analysis (35), attempts to predict the presence of chromosomal aberrations through AI tools from scanned H&E slides is still substantially lacking. However, the field is promising, as can be demonstrated by the possibility of detection of some of these fusions in other districts, such as in thyroid cancer. Indeed, starting from a cohort of 802 thyroid cancer H&E stained WSIs, of which 23 belong to tumors with NTRK fusions, a proposed CNN model to predict NTRK status demonstrated an AUC of more than 0.8 on images from the same cohort that was used for training (45). However, the model performance dropped significantly in external cohorts, A further promising frontier for this approach is represented by the integrative way, coupling histology-based AI with information coming from other subspecialities (46,47). In this setting, some groups already demonstrated the capability of radiomics from computed tomography (CT) (48) or positron emission tomography/computed tomography (PET/CT) (49) coupled with clinicopathological data to predict the presence of ALK rearrangements, stressing once again the importance of the integrative role of pathology and the enormous potentialities of digital pathology (Figure 2).
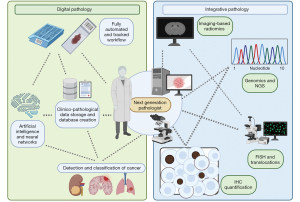
Limitations and challenges of the approach
The results obtained so far with the application of AI tools for the prediction of mutation/translocation in different types of cancer are exciting, but some drawbacks still exist and should be taken into account for the design of future and more rigorous studies. First of all, one of the limitations that often affect these studies is represented by the dimension of the used cohorts, especially the validation ones, often small and enriched in the most “common” histotypes of cancer. This last point may not ensure to cover the entire tumor heterogeneity that can be encountered in the clinical practice, potentially hampering the investigation of less frequent histologies, thus limiting the performances of the algorithms when applied to these rare tumors. Moreover, it should always be noted that the model is not a complete replacement for pathologists’ examination, which include the diversity and heterogeneity of tissues that pathologists typically inspect (e.g., inflammation, necrosis, and blood vessels). From the more “analytic” side of the AI algorithms, other specific challenges should be noted, starting from the need to normalize the stain of WSI (50), for which different methods exist (e.g., metric learning, domain agnostic learning, transfer learning or continual learning) that should be ideally tailored in future studies. Moreover, novel approaches in the field of explainable AI could help to better understand the visual features that drive the model decision, which could lead in the future to the identification of specific morphological aspects that can predict the underlying genetic landscape even for the human eye. Finally, the segmentation process is still quite heterogeneous among the different studies, with some authors performing a manual ROIs identification, which is time-consuming and prone to human errors. From these considerations, as compared to studies based on the automatic segmentation (17,20), those using a “manual” approach (18,19) could potentially be affected by some bias, as the “supervised” character of the analysis could affect the final results.
Translating AI tools in the routine clinical practice
In order to be able to predict genetic abnormalities for routine clinical use, the results of these studies should follow the requirements dictated by the regulatory authorities. In this direction, in order to be approved as in vitro diagnostic tools by the Food and Drug Administration (FDA) (51), such AI-based devices should follow strict methodological recommendations that can be summarized as follows:
- The technical performance assessment and study protocols (e.g., ROI localization study), as well as results used to assess the device output(s) (e.g., image overlays, image heatmaps, etc.), should be clearly declared and follow the most rigorous evidences;
- The training dataset must include cases representing different pre-analytical variables representative of the conditions likely to be encountered when used as intended (e.g., fixation type and time, histology slide processing techniques, multiple sites, patient demographics, challenging diagnostic cases especially when dealing with rare genetic variants);
- The number of WSI in an independent validation dataset must be appropriate to demonstrate device accuracy in detecting and localizing ROIs on scanned WSI, and must include subsets clinically relevant to the intended use of the device.
Another critical point that can significantly affect the performances of such AI predictive tools is represented by the size and number of tiles/patches automatically retrieved from the WSI by the algorithm during the training phase of the study. Recently, breaking WSIs into many smaller manageable image patches for high-throughput sampling have allowed investigators to overcome old AI approaches that only analyzed small ROIs and were unable to deal with large WSI. While increasing image patch size for training may lead to improved algorithm performance (52), this also could increase computation time and cost. Thus, independently from the actual number of patches used, the most important step is to ensure a good representation of the diversity of samples/pictures in the training set, because there can be substantial variance present within a single target class. Regarding the dimension single patches and magnification of the WSI employed, the situation is quite heterogeneous among the studies analysed in the present review, with some dealing with scanned slides at 20× (17,19,26,42) and others at 40× (31), with extracted regions of different size at 0.5 µm/px or 0,24 µm/px (MPP): 2,000×2,000 (31), 1,500×1,500 (19), 512×512 px (18,27,42), 360×360 (23) and 256×256 (17). However, all these studies share a common approach based on the exclusion of regions affected by processing artifacts or avoiding patches with a low amount of information (e.g., all the tiles with >50% of the surface covered by background, namely with values below 220 in the RGB color space). These methods seem to agree with those proposed in the datasheets of several diagnostic in vitro devices already approved by the principal regulatory authorities (51) that are already in use in clinical practice to morphologically detect tumor areas in WSIs. Finally, recent experiences highlighted the possibility of performing an annotation-free WSI training approach that does not require the detection of patches/tiles, specifically tested on lung cancer specimens, that would further simplify the workflow and reduce the variability among studies (53). The progressive clarification and standardization of these technical aspects is contributing to the significant increase in the number of trials aimed at applying AI-tools for cancer prediction. In particular, ongoing studies (ClinicalTrials.gov Identifier: NCT04217044) are evaluating the possibility to predict the most common genetic alterations of gliomas (1p/19q co-deletion, MGMT methylation, IDH and TERTp mutations, etc.) with AI from routine H&E stains. The subsequent step of this dramatic transformation will be the implementation in the routine practice of such tools, to be utilised by pathologists and oncologists which are not always familiar with complex informatics and AI. Thus, the future challenge will be the complete integration of these predictive algorithms in the currently employed automated workflows (3), as already experienced for diagnostic AI-based tools in clinical-grade computational pathology (14,54).
Conclusions
Digital pathology is becoming a valuable tool to predict gene aberrations in different types of tumors, with a promising role in lung cancer. What is truly changing the paradigm of molecular testing from a manual to a fully automated approach is probably represented by the introduction of digital scanning systems for IHC and FISH analysis, whereas the implementation of AI in this setting, even if promising, still requires further investigation.
Acknowledgments
We would like to thank Dr. Umberto Malapelle and Dr. Pasquale Pisapia from the University of Naples Federico II for their support in the creation of the iconography of this manuscript.
Funding: None.
Footnote
Provenance and Peer Review: This article was commissioned by the Guest Editors (Fabrizio Tabbò, Umberto Malapelle, Maria Lucia Reale, and Angela Listì) for the series “How to Detect and Treat NSCLC Patients With Oncogenic Fusions” published in Precision Cancer Medicine. The article has undergone external peer review.
Reporting Checklist: The authors have completed the Narrative Review reporting checklist. Available at https://pcm.amegroups.com/article/view/10.21037/pcm-21-56/rc
Conflicts of Interest: All authors have completed the ICMJE uniform disclosure form (available at: https://pcm.amegroups.com/article/view/10.21037/pcm-21-56/coif). The series “How to Detect and Treat NSCLC Patients With Oncogenic Fusions” was commissioned by the editorial office without any funding or sponsorship. The authors have no other conflicts of interest to declare.
Ethical Statement: The authors are accountable for all aspects of the work in ensuring that questions related to the accuracy or integrity of any part of the work are appropriately investigated and resolved.
Open Access Statement: This is an Open Access article distributed in accordance with the Creative Commons Attribution-NonCommercial-NoDerivs 4.0 International License (CC BY-NC-ND 4.0), which permits the non-commercial replication and distribution of the article with the strict proviso that no changes or edits are made and the original work is properly cited (including links to both the formal publication through the relevant DOI and the license). See: https://creativecommons.org/licenses/by-nc-nd/4.0/.
References
- L'Imperio V, Gibilisco F, Fraggetta F. What is Essential is (No More) Invisible to the Eyes: The Introduction of BlocDoc in the Digital Pathology Workflow. J Pathol Inform 2021;12:32. [Crossref] [PubMed]
- Fraggetta F, Garozzo S, Zannoni GF, et al. Routine Digital Pathology Workflow: The Catania Experience. J Pathol Inform 2017;8:51. [Crossref] [PubMed]
- Fraggetta F, Caputo A, Guglielmino R, et al. A Survival Guide for the Rapid Transition to a Fully Digital Workflow: The "Caltagirone Example". Diagnostics (Basel) 2021;11:1916. [Crossref] [PubMed]
- L'Imperio V, Brambilla V, Cazzaniga G, et al. Digital pathology for the routine diagnosis of renal diseases: a standard model. J Nephrol 2021;34:681-8. [Crossref] [PubMed]
- Griffin J, Treanor D. Digital pathology in clinical use: where are we now and what is holding us back? Histopathology 2017;70:134-45. [Crossref] [PubMed]
- Evans AJ, Brown RW, Bui MM, et al. Validating Whole Slide Imaging Systems for Diagnostic Purposes in Pathology: Guideline Update From the College of American Pathologists in Collaboration With the American Society for Clinical Pathology and the Association for Pathology Informatics. Arch Pathol Lab Med 2021; [Epub ahead of print].
- Fraggetta F, L'Imperio V, Ameisen D, et al. Best Practice Recommendations for the Implementation of a Digital Pathology Workflow in the Anatomic Pathology Laboratory by the European Society of Digital and Integrative Pathology (ESDIP). Diagnostics (Basel) 2021;11:2167. [Crossref] [PubMed]
- Salto-Tellez M, Maxwell P, Hamilton P. Artificial intelligence-the third revolution in pathology. Histopathology 2019;74:372-6. [Crossref] [PubMed]
- Holzinger A, Malle B, Kieseberg P, et al. Machine Learning and Knowledge Extraction in Digital Pathology Needs an Integrative Approach. Towards Integrative Machine Learning and Knowledge Extraction 2017:13-50. Available online:
10.1007/978-3-319-69775-8_2 10.1007/978-3-319-69775-8_2 - Bera K, Schalper KA, Rimm DL, et al. Artificial intelligence in digital pathology - new tools for diagnosis and precision oncology. Nat Rev Clin Oncol 2019;16:703-15. [Crossref] [PubMed]
- Fraggetta F, Pantanowitz L. Going fully digital: utopia or reality? Pathologica 2018;110:1-2. [PubMed]
- Sudharshan PJ, Petitjean C, Spanhol F, et al. Multiple instance learning for histopathological breast cancer image classification. Expert Syst Appl 2019;117:103-11. [Crossref]
- Xu Y, Zhu JY, Chang EI, et al. Weakly supervised histopathology cancer image segmentation and classification. Med Image Anal 2014;18:591-604. [Crossref] [PubMed]
- Campanella G, Hanna MG, Geneslaw L, et al. Clinical-grade computational pathology using weakly supervised deep learning on whole slide images. Nat Med 2019;25:1301-9. [Crossref] [PubMed]
- Ehteshami Bejnordi B, Veta M, Johannes van Diest P, et al. Diagnostic Assessment of Deep Learning Algorithms for Detection of Lymph Node Metastases in Women With Breast Cancer. JAMA 2017;318:2199-210. [Crossref] [PubMed]
- Sallman DA, Padron E. Integrating mutation variant allele frequency into clinical practice in myeloid malignancies. Hematol Oncol Stem Cell Ther 2016;9:89-95. [Crossref] [PubMed]
- Chen M, Zhang B, Topatana W, et al. Classification and mutation prediction based on histopathology H&E images in liver cancer using deep learning. NPJ Precis Oncol 2020;4:14. [Crossref] [PubMed]
- Tsou P, Wu CJ. Mapping Driver Mutations to Histopathological Subtypes in Papillary Thyroid Carcinoma: Applying a Deep Convolutional Neural Network. J Clin Med 2019;8:1675. [Crossref] [PubMed]
- Kather JN, Pearson AT, Halama N, et al. Deep learning can predict microsatellite instability directly from histology in gastrointestinal cancer. Nat Med 2019;25:1054-6. [Crossref] [PubMed]
- Yamashita R, Long J, Longacre T, et al. Deep learning model for the prediction of microsatellite instability in colorectal cancer: a diagnostic study. Lancet Oncol 2021;22:132-41. [Crossref] [PubMed]
- Wang ZY, Chen Z. Acute promyelocytic leukemia: from highly fatal to highly curable. Blood 2008;111:2505-15. [Crossref] [PubMed]
- Mosleh M, Mehrpouri M, Ghaffari S, et al. Report of a new six-panel flow cytometry marker for early differential diagnosis of APL from HLA-DR negative Non-APL leukemia. Scand J Clin Lab Invest 2020;80:87-92. [Crossref] [PubMed]
- Sidhom JW, Siddarthan IJ, Lai BS, et al. Deep learning for diagnosis of acute promyelocytic leukemia via recognition of genomically imprinted morphologic features. NPJ Precis Oncol 2021;5:38. [Crossref] [PubMed]
- Xie Y, Pittaluga S, Jaffe ES. The histological classification of diffuse large B-cell lymphomas. Semin Hematol 2015;52:57-66. [Crossref] [PubMed]
- Ambrosio MR, Lazzi S, Bello GL, et al. MYC protein expression scoring and its impact on the prognosis of aggressive B-cell lymphoma patients. Haematologica 2019;104:e25-8. [Crossref] [PubMed]
- Swiderska-Chadaj Z, Hebeda K, van den Brand M, et al. Predicting MYC translocation in HE specimens of diffuse large B-cell lymphoma through deep learning. Medical Imaging 2020: Digital Pathology. 2020. Available online:
10.1117/12.2549650 10.1117/12.2549650 - Swiderska-Chadaj Z, Hebeda KM, van den Brand M, et al. Artificial intelligence to detect MYC translocation in slides of diffuse large B-cell lymphoma. Virchows Arch 2021;479:617-21. [Crossref] [PubMed]
- Kluk MJ, Ho C, Yu H, et al. MYC Immunohistochemistry to Identify MYC-Driven B-Cell Lymphomas in Clinical Practice. Am J Clin Pathol 2016;145:166-79. [Crossref] [PubMed]
- Sukov WR, Hodge JC, Lohse CM, et al. TFE3 rearrangements in adult renal cell carcinoma: clinical and pathologic features with outcome in a large series of consecutively treated patients. Am J Surg Pathol 2012;36:663-70. [Crossref] [PubMed]
- Magers MJ, Udager AM, Mehra R. MiT Family Translocation-Associated Renal Cell Carcinoma: A Contemporary Update With Emphasis on Morphologic, Immunophenotypic, and Molecular Mimics. Arch Pathol Lab Med 2015;139:1224-33. [Crossref] [PubMed]
- Cheng J, Han Z, Mehra R, et al. Computational analysis of pathological images enables a better diagnosis of TFE3 Xp11.2 translocation renal cell carcinoma. Nat Commun 2020;11:1778. [Crossref] [PubMed]
- Owens MA, Horten BC, Da Silva MM. HER2 amplification ratios by fluorescence in situ hybridization and correlation with immunohistochemistry in a cohort of 6556 breast cancer tissues. Clin Breast Cancer 2004;5:63-9. [Crossref] [PubMed]
- Wolff AC, Hammond ME, Hicks DG, et al. Recommendations for human epidermal growth factor receptor 2 testing in breast cancer: American Society of Clinical Oncology/College of American Pathologists clinical practice guideline update. J Clin Oncol 2013;31:3997-4013. [Crossref] [PubMed]
- van der Logt EM, Kuperus DA, van Setten JW, et al. Fully automated fluorescent in situ hybridization (FISH) staining and digital analysis of HER2 in breast cancer: a validation study. PLoS One 2015;10:e0123201. [Crossref] [PubMed]
- Chea V, Pleiner V, Schweizer V, et al. Optimized workflow for digitalized FISH analysis in pathology. Diagn Pathol 2021;16:42. [Crossref] [PubMed]
- Lee B, Lee T, Lee SH, et al. Clinicopathologic characteristics of EGFR, KRAS, and ALK alterations in 6,595 lung cancers. Oncotarget 2016;7:23874-84. [Crossref] [PubMed]
- Warth A, Penzel R, Lindenmaier H, et al. EGFR, KRAS, BRAF and ALK gene alterations in lung adenocarcinomas: patient outcome, interplay with morphology and immunophenotype. Eur Respir J 2014;43:872-83. [Crossref] [PubMed]
- Saric M, Russo M, Stella M, et al. CNN-based Method for Lung Cancer Detection in Whole Slide Histopathology Images. The 4th International Conference on Smart and Sustainable Technologies (SpliTech), 2019. Available online:
10.23919/SpliTech.2019.8783041 10.23919/SpliTech.2019.8783041 - Wei JW, Tafe LJ, Linnik YA, et al. Pathologist-level classification of histologic patterns on resected lung adenocarcinoma slides with deep neural networks. Sci Rep 2019;9:3358. [Crossref] [PubMed]
- Yu KH, Zhang C, Berry GJ, et al. Predicting non-small cell lung cancer prognosis by fully automated microscopic pathology image features. Nat Commun 2016;7:12474. [Crossref] [PubMed]
- Pham HHN, Futakuchi M, Bychkov A, et al. Detection of Lung Cancer Lymph Node Metastases from Whole-Slide Histopathologic Images Using a Two-Step Deep Learning Approach. Am J Pathol 2019;189:2428-39. [Crossref] [PubMed]
- Coudray N, Ocampo PS, Sakellaropoulos T, et al. Classification and mutation prediction from non-small cell lung cancer histopathology images using deep learning. Nat Med 2018;24:1559-67. [Crossref] [PubMed]
- Conde E, Suárez-Gauthier A, Benito A, et al. Accurate identification of ALK positive lung carcinoma patients: novel FDA-cleared automated fluorescence in situ hybridization scanning system and ultrasensitive immunohistochemistry. PLoS One 2014;9:e107200. [Crossref] [PubMed]
- Hernandez S, Conde E, Prieto M, et al. P1.02-043 multiplexed fish (ALK/ROS1, Ret, NTRK1) in lung adenocarcinomas: Novel dual ALK/ros1 probe and automated scanning system. J Thorac Oncol 2017;12:S513-4. [Crossref]
- Höhne J, de Zoete J, Schmitz AA, et al. Detecting genetic alterations in BRAF and NTRK as oncogenic drivers in digital pathology images: towards model generalization within and across multiple thyroid cohorts. Proceedings of Machine Learning Research 2021;156. Available online: https://proceedings.mlr.press/v156/hohne21a/hohne21a.pdf
- Le NQK, Hung TNK, Do DT, et al. Radiomics-based machine learning model for efficiently classifying transcriptome subtypes in glioblastoma patients from MRI. Comput Biol Med 2021;132:104320. [Crossref] [PubMed]
- Le NQK, Kha QH, Nguyen VH, et al. Machine Learning-Based Radiomics Signatures for EGFR and KRAS Mutations Prediction in Non-Small-Cell Lung Cancer. Int J Mol Sci 2021;22:9254. [Crossref] [PubMed]
- Song Z, Liu T, Shi L, et al. The deep learning model combining CT image and clinicopathological information for predicting ALK fusion status and response to ALK-TKI therapy in non-small cell lung cancer patients. Eur J Nucl Med Mol Imaging 2021;48:361-71. [Crossref] [PubMed]
- Chang C, Sun X, Wang G, et al. A Machine Learning Model Based on PET/CT Radiomics and Clinical Characteristics Predicts ALK Rearrangement Status in Lung Adenocarcinoma. Front Oncol 2021;11:603882. [Crossref] [PubMed]
- Janowczyk A, Basavanhally A, Madabhushi A. Stain Normalization using Sparse AutoEncoders (StaNoSA): Application to digital pathology. Comput Med Imaging Graph 2017;57:50-61. [Crossref] [PubMed]
- Raciti P, Sue J, Ceballos R, et al. Novel artificial intelligence system increases the detection of prostate cancer in whole slide images of core needle biopsies. Mod Pathol 2020;33:2058-66. [Crossref] [PubMed]
- Bejnordi BE, Zuidhof G, Balkenhol M, et al. Context-aware stacked convolutional neural networks for classification of breast carcinomas in whole-slide histopathology images. J Med Imaging (Bellingham) 2017;4:044504. [Crossref] [PubMed]
- Chen CL, Chen CC, Yu WH, et al. An annotation-free whole-slide training approach to pathological classification of lung cancer types using deep learning. Nat Commun 2021;12:1193. [Crossref] [PubMed]
- Fraggetta F. Clinical-grade Computational Pathology: Alea Iacta Est. J Pathol Inform 2019;10:38. [Crossref] [PubMed]
Cite this article as: Beretta C, Ceola S, Pagni F, L’Imperio V. The role of digital and integrative pathology for the detection of translocations: a narrative review. Precis Cancer Med 2022;5:16.