Precision medicine and future of cancer treatment
The concept of precision medicine can be arguably dated back into the 1960s when such medicines were introduced for the first time through the concept encompassing the terms pharmacogenetics/pharmacogenomics by Evans and Clark (1). With the advent of high-throughput data generated through next generation sequencing (NGS) technologies, the term started metamorphosing from ‘Systems medicine’ or ‘Systems biomedicine’ to ‘Precision medicine’ and ‘Personalized medicine’ (1-5). Later, with more genome level data analysis and systems biological data pouring in, terms like ‘Genomic-era medicine’ (6), ‘Predictive, preventative, personalized and participatory (P4) medicine’ (7), ‘Me medicine’ (8), ‘P4 systems medicine’ (9) and ‘Computational systems biomedicine’ (10) were referred to the same concept. Essentially, this involves intervention therapies encompassing the impact of any genes of individuals and their exposures to lifestyle and environment (11). Despite almost similar in thoughts, ‘Personalized medicine’ and ‘Precision medicine’ differ vividly (12). In the words of Ginsburg and Phillips, the former refers to an approach to patients that considers their genetic make-up but with attention to their preferences, beliefs, attitudes, knowledge and social context. In contrast, the latter ‘describes a model for health care delivery that relies heavily on data, analytics, and information’ (12). The main concept of precision medicine, however, revolves around the rapid identification of new drug targets and an interpretation of the patient-specific mechanisms causing or contributing to the genetic variation of the diseases (13). This concept has revolutionized the medical treatment of many complex diseases. Mentionable among them is cancer, which had been proliferating at the mercy of unimpressive efficacy of earlier developed drugs, mainly due to its heterogeneous causes (13). To this end, side-effects, arising from the pleiotropic nature of genes, has also been taken into account while addressing the pressing need of identifying new cancer drug targets (14).
While research continues to identify novel cancer protein targets, there is a necessity for further therapeutic exploration. Moreover, advances in high-throughput genomics and proteomics have vastly increased the scope to better understand such cancer pathogenesis cropping up from the complex interactions between the genes and the environment. Such complex interactions between the genes can give rise to pleiotropism wherein a single gene affects a number of phenotypic traits in the same organism. Thus, the protein products of these genes, if targeted indiscriminately through different drugs, could then be affected. In this context, a detailed holistic approach has been attempted for identifying novel cancer genes and proteins which upon drug targeting, would give rise to side effects (14). However, to cure/treat complex diseases like cancer, it is essential that we identify and target their root causes. This, in turn, requires a detailed exploration at genomic level to identify and validate mutations and/or other genomic/transcriptomic changes specific to a cancer in general or a subgroup of patients within a cancer. Essentially, high frequency of chromosomal aberrations has been observed in different cancer types. These include translocations of chromosomes, amplification and deletion events, mutations as well as elongation of telomeres (15). The large-scale genomic changes, at both the DNA sequence and chromosome level, are not only seen in cancer patients at diagnosis, but continue to evolve with time. This indicates a genomic instability, a fundamental feature of most human cancers, and contributes to the development and progression of malignant phenotypes (15). A pertinent role of elevated levels of recombinase (RAD51) and homologous recombination activity, in such aforementioned aberrations in cancer, has been explored in detail (15-17). Other instances of genomic instability, arising from impaired DNA double strand break (DSB) repair, has been shown to be caused by SPOP (Speckle-type POZ protein) mutation in prostate cancer (18). Further insights on clonal mutations of proteins like L1 cell adhesion molecule (L1CAM) and APOBEC are obtained for urothelial carcinoma (19). Increased mutations and/or changes in the expression of these type of genes may also contribute to cancer multidrug resistance, thereby posing plausible hindrances towards the development of precision cancer medicines.
Due to a new open data initiative over the last few decades, a wide range of clinical and basic biological research data have become available. These range from genomic, transcriptomic and proteomic to metabolomic and interactomic data. Thus, with an ultimate goal to effectively manage this huge deluge of information, a new field of study has emerged to give a global perspective (20,21). Coined as ‘Systems Biology’, this would aid in understanding the diseases per se, which themselves serve as a conglomerate of multitudes of molecular entities regulated through different networks and belonging to various affected pathways (21,22). Thus, a systems biological approach of the diseases is considered to give a bird’s eye view at the systems level of diseases. Essentially, this would help in identifying different diagnostic biomarkers and developing intervention therapies through discovery, optimization and combinations of the drugs (20,21). Table 1 deals with such huge explosion of experimental data from different biotechnological procedures and maintained in different types of databases.
Table 1
Representative databases | Experimental data | Biotechnologies |
---|---|---|
Genbank, DDBJ, Ensembl | DNA sequences, exome sequences, genomes, genes | DNA-seq, NGS |
GEO, Expression Atlas | Gene expression levels, microRNA levels, transcripts | Microarray, RNA-seq, Fluorescent in situhybridization, RT-PCR |
GPMdb, PRIDE, Human Protein Atlas | Protein concentration, phosphorylation | MS, iTRAQ |
HMDB, GMD | Metabolite levels | LC-MS, GC-MS, NMR |
GEO, TRANSFAC, JASPAR, ENCODE, modENCODE | Protein-DNA interactions, transcript factor binding sites | ChIP-chip, ChIP-seq |
StarBase, miRTarbase | MicroRNA-mRNA regulations | CLIP-seq, PAR-CLIP, iCLIP |
HPRD, BioGRID, DIP, IntAct and MINT, CCSB interactome database, STRING | Protein-protein interactions | Y2H, AP/MS, MaMTH, maPPIT |
RegPhos, PhosphoPOINT | Kinase-substrate interactions | Protein microarray |
HPRD, BioGRID | Genetic interactions | SGA, E-MAP, RNAi, CRISPR-Cas9 |
GWAS Catalog, GWASdb, GTEx, dbGAP, dbSNP, HGMD | GWAS lod, eQTL, aberrant SNPs, Genome-wide association studies typically conducted on individuals who have had a serious ADR, or who have serious ADRs withing their family’ | SNP genotyping array, CNV, Whole exome sequencing, Whole genome sequencing |
KEGG, ConsensusPathDB, BioCart, Pathway Commons, MsigDB, Reactome, BiGG | Signalling pathways, metabolic pathways, molecular signatures | LUMIER, data integration |
DrugBank, STITCH, Chembl, Matador, PDSP | Drug-target interaction | Affinity chromatography with mass spectrometry, SPR |
Gene overexpression | cDNA/ORFs | |
Drug efficacy | HTS, MTT assay | |
EMR/HER | Digitalization | |
Drug_ADR DB | ||
Offsides | Database of significant drug-effect associations | – |
SIDER | Drug side effect reference with ADR information extracted from public information and product labels | – |
CTD | Comparative Toxicogenomics Database | – |
ATC | Anatomical Therapeutic Chemical (ATC) | – |
Chemical structure DB | ||
PubChem compound | Repository of validated chemical information | – |
ZINC | Database of commercially available compounds for virtual screening | – |
Connectivity map | Collection of transcriptional expression data from drug-treated cultured human cells | – |
Drug-drug interaction (DDI) DB | ||
Two sides | Database of significant drug-drug effect associations | – |
INDI | Inferring drug interactions | – |
Drugs.com | Database of DDIs | – |
Gene function DB | ||
GO | Gene ontology | – |
GeneCards | Comprehensive database in genes and gene function | – |
OMIM | Reference for human genes and genetic disorders (Mendelian and non-Mendelian diseases | – |
Protein structure/sequence | ||
PDB | Protein Data Bank | – |
Uniprot | UniProtKB/Swiss-Prot | – |
Protein-ADR DB | ||
DART | Developmental and reproductive toxicology | – |
DITOP | Drug-induced toxicology-related proteins | – |
Protein-gene interaction DBs | ||
TRED | TRED Transcriptional Regulatory Element Database | – |
ChEA | Chip Enrichment Analysis Database | – |
Postmarket reporting systems (PMRS) | ||
FAERS | Registry of adverse event and medication error reports sent to the FDA and Centres for Disease Prevention Control l(CDC) | – |
VAERS | Registry of vaccine-related adverse events sent to the FDA and CDC | – |
MedEffect Canada | Registry of adverse event and medication error reports from Canada | – |
Disease terminologies | ||
ICD | International Classification for Disease, a hierarchical disease classification for billing purposes | – |
MedDRA | Specialized medical terminology designed for the pharmaceutical industry | – |
UMLS | Unified Medical Language System, and integrated terminology system | – |
MeSH | Medical Subject Heading vocabulary | – |
SNOMED | Systemized Nomenclature for Medicine, an ontology for medical terms | – |
Clinical trials | Registry and results repository for clinical trials | – |
Electronic health records (EHRs) | ||
Billing data | Medical information collected at the point-of-care by healthcare providers. Data collected primary for insurance and billing purposes. Data can be reused for pharmacovigilance | – |
Clinica narratives | Clinical notes taken at the point-of-care by clinicians and healthcare providers. Data can be reused for pharmacovigilance. | – |
Surveys | Questionnaires sent to providers regarding medications, given that result in ADBs, and other comorbities that patients were on | – |
Metabolic data | Metabolic studies that find specific metabolic profiles associated with serious ADR’s and ADR outcomes | – |
The first layer, stated as “Cause”, shows the different levels of modifications used by scientists to explore and develop precision medicine for cancer through systems biological approaches. The second layer shows the “Effect” of such explorations by the scientists leading to the deluge of data and techniques. The third layer delineates the tools used by the scientists to explore and generate the concepts underlying precision medicine. This is reflected as the “Link”.
In one of the attempts to provide a systems level model for breast cancer, Carels et al. used different cell lines having different histological subtypes to understand the state of the hormonal receptors (23). These are the estrogen receptor (ER), progesterone receptor (PR), and human epidermal growth factor receptor 2 (HER2, also known as ERBB2) which can give an indication of the patient specific therapies (23). With a temporal data of the post personalized treatments, they have provided a Kaplan-Meier graph illustrating the relative 5-year survival of the patients (23). Delving deep into the molecular time of the spread of the breast cancer, they have delineated a modified version of the tumour heterogeneity evolution, encompassing genomic instability and clonal expansion (23). Further utilisation of patient-derived tumour samples has been attempted to aid in identifying effective drugs and/or combinations thereof, for further clinical trial assessments and personalized therapeutic treatments (11). For this, Pauli et al. have integrated the whole-exome sequences with the 56 tumor-derived organoid cultures and 19 patient-derived xenograft (PDX) models of uterine malignancies and colon cancers (11). Furthermore, concepts of integrating huge accessible biological data of the traditional natural products with those of the synthetic western medicines have been proposed as an alternative and effective strategy towards cancer precision medicines. This has become a necessity owing to the challenges posed by cell subpopulations, differing phenotypically and genetically, thereby giving rise to tumour heterogeneity (24). With a network pharmacological approach of leukaemia cells affected by treatment with neoambrosin or damsin from Ambrosia maritima, Efferth et al. have analysed to portray the three different gene targets. These are the master, responder and effector for all natural products, therapeutic antibodies and synthetic small molecules (24). The main reason for such integration, they believe, is possible due to the fact that natural products serve as the repertoire of mixtures with multi-specificity such that they can aim different targets simultaneously (24). The underlying concept in utilizing such natural products is the essence of polypharmacy which is being mostly used for elderly patients (25,26). However, there can be risk factors associated with side-effects of multiple drugs as reported by Finkelstein et al. for all patients put on a drug regimen of 17–26 drugs at a time (27). Despite being studied in a completely different scenario, with patients having concurrent chronic heart and lung diseases, this showed a complicated drug-drug and drug-gene interactions, thereby exemplifying the risks (26). Furthermore, the condition of polypharmacy necessitates the study of precision medicines for identifying the adverse reactions of several small molecules as drugs. Commonly referred to as ADRs (adverse drug reactions), many small molecules cause unintended side-effects which vary widely in their mechanism of actions and severity of the reactions affecting a diverse range of populations (27). The assessment of such ADRs largely depends on the reports generated from size-limiting clinical trials and biased post market survey which predisposes the patients towards insufficient knowledge-based risks (27). In an attempt to overcome such risks associated in unravelling the ADRs, systems pharmacology has been used by researchers for some time now. This entails a detailed network based analysis incorporating the interactions between the chemical and biochemical entities like the drugs and food molecules of the diet and biological entities encompassing the genes and proteins. In their comprehensive review, Boland et al. has elucidated information from a bipartite graph indicating the connections between all of these components as used by researchers of systems pharmacology (27).
With the advent of time, treatment of cancer has undergone several changes tailored to the need of catering to the mass, precisely and effectively. This encompassed the identification of specific gene mutations and presence or absence of specific gene functions causing uncontrolled proliferation at the site of origin and/or gross chromosomal rearrangements. However, despite such efforts, the intratumoral heterogeneity and ongoing genomic evolution make a complication for the identification of patients which may benefit from specific cancer treatment/s (28). Epigenetic modifiers which play crucial role in the maintenance of chromosome structure and function, have also been started to be targeted by emerging technologies (28). Such strategies attempt to integrate the concept of malignant transformation being modulated by interactions evident from genetic and epigenetic modifications (28). Identification of genetic and epigenetic mechanisms of malignant transformation has accelerated the process of discovering the genetic variants responsible for the disease and development of drugs targeting them. However, a large number of genetic variants, including rare mutations (minor allele frequency, MAF <1%) besides polymorphisms (MAF >1%), ca modulates the truly personalized prediction of drug responses (29). Encompassing such somatic variants besides the germline polymorphisms in the tumor genome as directives for pharmacogenetics-informed prescription have started to be utilised for certain drug/gene pairs (29). Revisiting them from a Brazilian perspective (29), Suarez-Kurtz reviewed and substantiated the beneficial effects of such drug/gene pairs of irinotecan/UGT1A1, tamoxifen/CYP2D6, thiopurines/TPMT and fluoropyrimidines/UGT1A1 as directed by the Clinical Pharmacogenetics Implementation Consortium and/or the Dutch Pharmacogenetic Working Group (30).
The above discussion, thus, takes us to the juncture to understand that the integration of data science and analytics onto pharmacogenetics and/or pharmacogenomics is imperative to pave the way for precision cancer medicine. This, in turn, would shape up the society having proper research background developed with optimized tools and information to provide help and care for improved cancer patient outcomes. This can even lead to the development of new economic policy and agenda both locally and globally until such new paradigm of health care is adopted (8).
The direct linkage between human genetics and drug response, in terms of their co-variation, have been put together in the largest manually curated database called the Pharmacogenomics Knowledge Base (PharmGKB) (31). However, due to the vastness, dynamicity and complexity of the biomedical terms and knowledge, an extraction of such published facts, to transform into meaningful and translatable information, is a mammoth task (32). Thus, to aim towards precision medicine, the earliest of the methods, adopted a concept of connecting the genes with the drugs relying on machine learning, besides statistics (33). In fact, statistical methods to determine co-occurrence along with a typical machine learning method, namely Natural Language Processing (NLP) have been used by Chang and Altman (34) to classify the drug-gene pairs extracted from literature. Later, Garten and Altman extracted the relationships between the specific concepts of Phramacogenomics (PGx) through a text-mining tool named Pharmspresso (35). Moreover, based on the earlier reported co-occurrence statistics, Theobald et al. created conditional probability tables to transform them into n-way Bayesian networks to analyze the relationship between the biological entities (36). Nevertheless, development of the NLP system continued to be in practice to uncover the drug-gene relationships from co-occurrence in MEDLINE citations and abstracts through Enhanced SemRep, Syntactic Dependency Structure and other conditional approaches (37-39).
With a detailed focus towards precision cancer medicine, efforts have also been made to rank the genes of PGx importance in order of their potential drug-specific relevance (40,41). In fact, a semi-supervised iterative searching and ranking (bootstrapping) algorithm has been reported with an aim to prioritize the PGx genes (32,42). Using 20 million MEDLINE abstracts and PGx specific genes, the algorithm reached a precision of 0.219 after two iterations which increased to 0.561 in the end, for top ranked pairs. This was way above in terms of precision, recall or F1 (precision: 0.251 vs. 0.152, recall: 0.396 vs. 0.856 and F1: 0.292 vs. 0.254) when compared to non-PGx-specific seeds or even co-occurrence of MEDLINE terms (32,42). One might like to revisit the terms called precision, recall and F1. The first of these refers to the ratios of true positives over total positives including false, while the second refers to the true positives over actual positives including false negatives. Finally, the last one stands for a weighted average of precision over recall. A strong correlation was achieved when the predicted drug-gene pairs were compared with the drug adverse events (42).
While drug-gene relationships are being investigated to rank them in order of their relevance in treatment, efforts have been taken to have a detailed mapping of the cancer cell evolution, commonly referred to as clonal evolution, incorporating the tumour DNA purity and cancer cell ploidy (43). However, considering the tumour heterogeneity, which might develop owing to either progression or treatment, global optimization methods (44-47) might fail to measure the complexity of the underlying cellular population as they ignore the genomic diversity (43). Thus, a consideration of the three types of cell populations namely, non-tumour and tumour with and without deletions, contributing to the allelic frequency values of informative SNPs with a somatic deletion, helped researchers to come up with a local optimization method (43). Termed as CLONET (CLONality Estimate in Tumors), Prandi et al. developed the model using the probability distribution of the observed AFs, to compute the local admixture values for all deletions across the genome and uncover the selected lesions utilizing the whole genome sequencing (WGS) data of tumour samples comprising 21 lung adenocarcinomas (48), 24 metastatic melanomas (49) and 55 primary prostate cancers (50).
Further consideration of a combined effect of ‘omics’ data driven analyses, coupled with systems biology and mathematical modelling approaches, have been made in shifting the theranostic paradigm. Known as OncoTrack, the program aims to record the responses of individual samples of primary tumor, metastases and paired healthy tissue to drugs besides maintaining 3D cell cultures of tumors and mouse xenograft models (51). This is aided by the pre- and post-treatment analyses of the corresponding omics data comprising the whole genome, exome, transcriptome, methylome, and global proteomes of colon cancer patients (51). The whole concerted effect helps to predict the responses of the patients giving an idea of the drug resistance development in case of failures of such responses (51). Similar massive integration of genomic data driven initiatives like IGNITE have come into play to help build the network of multiple Electronic Health Records (52,53). Being established in 2013, the National Institutes of Health-funded initiative, IGNITE (Implementing GeNomics In pracTicE; www.ignite-genomics.org) Network, has a coordinating center which manages six projects for developing, investigating and disseminating genomic medicine practice models with clinical decision support (CDS). Some centres of IGNITE network (e.g., Vanderbilt University) maintain external link for the CDS (e.g., MyCancerGenome.org) (52).
Furthermore, cancer specific large scale big biomedical data integration has been in practice to analyze and provide a multifaceted user platform for learning and care decision making. One such is G-DOC Plus which incorporates more than 10,000 patients’ data from different public and private resources including the Gene Expression Omnibus (GEO), The Cancer Genome Atlas (TCGA), REpository for Molecular BRAin Neoplasia DaTa (REMBRANDT), caArray studies of lung and colon cancer, ImmPort and the 1000 genomes data sets (54). The tools incorporated in G-DOC Plus have been extensively used for generating hypothesis and validating them for cancer and non-cancer studies by exploring the somatic mutations and cancer MRI images through multi-omics analysis to aid into biomarker discovery besides providing knowledge based training in the related fields of bioinformatics, computational and sciences (54). Provision of web-based tool is also made available through IDICAP used for Integrating Drug Intervention based on Cancer Panel (55). Essentially, the tool links breast, ovarian and general cancer genes with drugs and clinical trials information from ClinicalTrials.gov and DrugBank besides integrating facts and figures related to drugs under trial and their locations, drugs with known targets of the queried genes, any known single nucleotide polymorphism (SNP) effects and other disease conditions (55,56). IDICAP has been claimed to be precise enough for indicating clinical trials and drugs compared to similar results from Drug Gene Interaction Database (DGIdb) or even ClinicalTrials.gov (55,57). All such large data integration tools take the whole initiative of precision cancer medicine, a step forward.
Taking the precision cancer medicine initiative from a multi-forked analytical approach to streamlined cases of network based analyses coupled with relevant bioinformatics pipelines, helped researchers to identify multi-target drug therapies and differentially prioritize them in triple negative breast cancer (TNBC) models and otherwise (58-61). Effectively starting with certain tumor type/subtype, Vitali et al. graph theoretically analysed the disease-specific Protein-Protein Interaction (PPI) network derived by combining different databases and knowledge repositories to identify a set of potentially interesting combinations of drug targets (58). Applying an approach based on matrix tri-factorization coupled with the available knowledge about the mechanisms of action of selected drugs, a number of in vitro experiments upon TNBC validated the method (58). In another study on TNBC models, a computational platform, entitled GenEx-TNBC, has been deployed through network-based approach to prioritize thousands of approved and experimental drugs for therapeutic potential against each molecular subtype of TNBC (59). Essentially, networks were constructed from patient-based and cell-line-based gene expression data and analysed for statistical coincidence with drug action networks stemming from known drug-protein targets (59). The approach via GenEx-TNBC successfully defined drugs and classified them for further in vitro testing so as to establish this platform as the first of its kind to associate drugs to diseases (59). Utilization of such known and predicted protein-protein interaction databases like STRING (62) and protein-drug interactions from STITCH (63), have enabled the construction of evidence networks based upon a statistical test to detect differential statistical dependencies namely Evaluation of Differential DependencY (EDDY) (60). EDDY has been represented in the form of a public portal EDDY-CTRP where the Cancer Therapeutics Response Portal (CTRP), a dataset with drug-response measurements for more than 400 small molecules, and RNAseq data of cell lines in the Cancer Cell Line Encyclopedia (CCLE) have been integrated (60). Tran et al. believes that their method of building evidence networks to find ~70% of drug-mediator pairs have the potential to provide important insights to drug sensitivity (60). In fact, utilization of several such molecular networks with proteins, drugs, and/or genes have enabled the researchers in the last decade to aid into identify potential targets and pathways through network topological analysis and other graph theoretical measures (61). One such work, reported by Ashraf et al., is a recent description where different centrality measures have been utilized to compare and contrast among them and identify targets without potential side-effects (14). Essentially, they have shown the effectiveness of eigenvector centrality (C) along with other network parameters like k-core (K) and functional modularity (F) to bring forth the KFC criterion as crucial to determine side-effects of marketed drugs reflected in Drugbank (14,56). Table 2 records several such gene-drug interactions as found from different specific tumor types.
Table 2
Gene symbol | Gene name | Effect of alteration | Major associations with specific tumor types | Implicated therapy |
---|---|---|---|---|
Protooncogenes and oncogenes | ||||
BCR-ABL | Breakpoint cluster region, Abelson murine leukemia viral oncogene homolog fusion protein | Compromises fidelity of DNA repair, deregulates proliferation, impairs apoptosis and differentiation | Chronic myelogenous leukemia | Imatinib, dasatinib, |
HRAS/KRAS | Harvey/Kristen rat sarcoma viral oncogene homolog | Constitutively activates MEK/ERK progrowth signalling | Non-small cell lung cancer | Salirasib |
BRAF | v-Raf murine sarcoma viral oncogene homolog B | Constitutively activates MEK/ERK progrowth signalling | Melanoma, V600E or V600K mutations | Vemurafenib, dabrafenib |
BCL2 | B-cell lymphoma/leukemia-2 | Impairs apoptosis | Leukemia, lymphoma, melanoma | Venetoclax |
Tumor suppressor genes | ||||
IDH1/2 | Isocitrate dehydrogenase | DNA hypermethylation, disrupts differentiation | Acute myeloid Leukemia (AML) | AG120,AG221, AG881 |
EZH2 | Enhancer of zeste 2 polycomb repressive complex 2 subunit | Inhibits apoptosis, silences by H3K27 trimethylation | Lymphoma | Tazemetostat |
DOTIL | DOT1-like histone H3K79 methyl transferase | Inhibits differentiation and apoptosis | Mixed-lineage leukemia | Pinometostat |
DNMT | DNA methyltransferase | Disrupts normal patterns of DNA methylation | Breast and colon cancers, glioma, AML | Azacytidine,decitabine |
HDAC | Histone deacetylases | Disrupts normal patterns of histone acetylation | Gastric, breast, colorectal cancers | Vorinostat, romidepsin |
Other targets | ||||
ER | Estrogen receptor | Sustains proliferative growth signals | Breast and Ovarian cancers | Tamoxifen |
CD20 | B-lymphocyte antigen, cluster of differentiation (CD) 20 | Supports B-cell activation and cell cycle progression | B-cell lymphomas | Rituximab |
ERBB2 (HER2/new) | Human epidermal growth factor receptor 2 | Sustains proliferative growth signals | Breast, ovarian, uterine and lung cancers | Trastuzumab |
PD1 | Programmed cell death protein 1(CD279) | Prevents activation of T-cells | Potentially targets all solid tumor | Nivolumab |
CTLA4 | Cytotoxic T-lymphocyte associated protein 4 | Prevents activation of T-cells | Potentially targets all solid tumor | Ipilimumab |
AP-1 | Activating protein 1 | Regulates gene expression controlling differentiation, proliferation and apoptosis | Colorectal cancer case study | Irbesartan (angiotensin II receptor antagonist) |
PML-RAR | Promelocytic leukemia, retinoic acid receptor alpha fusion gene | Inhibits granulocytic differentiation | Acute promyelocytic leukemia | Retinoic acid |
Biomarkers for clinical agents | ||||
APAF1 | Apoptotic protease activating factor 1 | Prevents apoptosis | Melanoma | Doxorubicin |
MGMT | O6-methylguaniine-DNA methyltransferase | Reverses DNA damage | Glioma | Alkylating agents |
When researchers worldwide are highly active to use several statistical and mathematical parameters and measures to analyse huge biological data for developing precision cancer medicine, bioinformatics programmers were not far behind to aid to their needs. As genomic aberrations, accompanying clinical and phenotypic features, are effectively identified through NGS, potential workflows and pipelines have started pouring in to identify potential gene targets and their corresponding drugs (64). Additionally, the R/Bioconductor package rDGIdb have enabled the R-users to have ready-made packages, with resources and tools, for dealing such NGS pipelines along with DGIdb, having drug-gene interaction from 15 different resources (57,64). Furthermore, another R package, namely the Cancer Variant Explorer (CVE), leverages the Oncotator and DGIdb, to provide variants within multiple or single tumour exomes so as to identify the driver mutations in a variant population along with their resistance mechanisms and in essence, assess the druggability of the targets (65,66). As per Mock et al, CVE enables the researcher to an explorative analysis of tissue specific networks for translational research studies (65). Last, but not the least, in an ongoing attempt to predict the drugs along with their targets, a new methodology of predicting them in a pairwise manner, has been adopted. This method is a Kernel-based machine learning approach and the multiple kernel learning (MKL) approach known as pairwiseMKL (67). In this method, Cichonska et al. showed its performance in accurately predicting two related tasks of anticancer efficacy of drug compounds across a large panel of cancer cell lines along with target profiles of anticancer compounds across their kinome-wide target spaces, using up to 167,995 bioactivity measurements and 3120 pairwise kernels (67).
In summary, the new technologies have emerged which allow evaluation of genetic, epigenetic and transcriptomic changes over whole genome (Figure 1). Moreover, with advances in bioinformatics, we can now integrate different types of data to identify genomic changes as well as underlying mutational processes in cancer (Figure 1). This has set the foundation for identification of better diagnostic/prognostic markers and therapeutic targets for specific cancers as well as for subgroups of patients within a cancer (Figure 1). Application of these technologies at individual patient level can potentially help in choosing the right drug, combination and/or strategy for that individual. Although it is still needed to remove/minimize some of the limitations associated with these technologies, they provide the bases for personalized and precise healthcare. Days are not far when network biology based predictive measures would lead the world to a completely new era of precision cancer medicine, tailor-made to fit the individual needs of fighting the deadly disease.
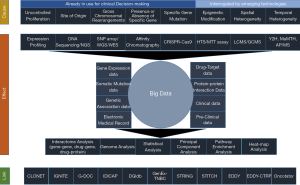
Acknowledgments
The authors acknowledge the support of Sunway University, Selangor, Malaysia for providing the computational facilities.
Funding: None.
Footnote
Provenance and Peer Review: This article was commissioned by the Guest Editor Masood A. Shammas for the series “Genomic Instability, Clonal Evolution and Oncogenesis” published in Precision Cancer Medicine. The article has undergone external peer review.
Conflicts of Interest: All authors have completed the ICMJE uniform disclosure form (available at http://dx.doi.org/10.21037/pcm.2019.09.01). The series “Genomic Instability, Clonal Evolution and Oncogenesis” was commissioned by the editorial office without any funding or sponsorship. The authors have no other conflicts of interest to declare.
Ethical Statement: The authors are accountable for all aspects of the work in ensuring that questions related to the accuracy or integrity of any part of the work are appropriately investigated and resolved.
Open Access Statement: This is an Open Access article distributed in accordance with the Creative Commons Attribution-NonCommercial-NoDerivs 4.0 International License (CC BY-NC-ND 4.0), which permits the non-commercial replication and distribution of the article with the strict proviso that no changes or edits are made and the original work is properly cited (including links to both the formal publication through the relevant DOI and the license). See: https://creativecommons.org/licenses/by-nc-nd/4.0/.
References
- Duffy DJ. Problems, challenges and promises: perspectives on precision medicine. Brief Bioinform 2016;17:494-504. [Crossref] [PubMed]
- Zhang Z, Zhao Z, Liu B, et al. Systems biomedicine: it’s your turn-recent progress in systems biomedicine. Quant Biol 2013;1:140-55. [Crossref]
- Kamada T. System biomedicine: a new paradigm in biomedical engineering. Front Med Biol Eng 1992;4:1-2. [PubMed]
- Wasi P. Human genomics: implications for health. Southeast Asian J Trop Med Public Health 1997;28:19-24. [PubMed]
- Langreth R, Waldholz M. New era of personalized medicine targeting drugs for each unique genetic profile. Oncologist 1999;4:426-7. [PubMed]
- Ashburn TT, Wilson SK, Eisenstein BI. Human tissue research in the genomic era of medicine: balancing individual and societal interests. Arch Intern Med 2000;160:3377-84. [Crossref] [PubMed]
- Hood L, Heath JR, Phelps ME, et al. Systems biology and new technologies enable predictive and preventative medicine. Science 2004;306:640-3. [Crossref] [PubMed]
- Dickenson D. Me medicine vs. we medicine: Reclaiming biotechnology for the common good. Columbia University Press, 2013.
- Cesario A, Auffray C, Russo P, et al. P4 medicine needs P4 education. Curr Pharm Des 2014;20:6071-2. [Crossref] [PubMed]
- Dubitzky W. Understanding the computational methodologies of systems biology. Brief Bioinform 2006;7:315-7. [Crossref] [PubMed]
- Pauli C, Hopkins BD, Prandi D, et al. Personalized in vitro and in vivo cancer models to guide precision medicine. Cancer Discov 2017;7:462-77. [Crossref] [PubMed]
- Ginsburg GS, Phillips KA. Precision medicine: From science to value. Health Aff (Millwood) 2018;37:694-701. [Crossref] [PubMed]
- Dugger SA, Platt A, Goldstein DB. Drug development in the era of precision medicine. Nat Rev Drug Discov 2018;17:183-96. [Crossref] [PubMed]
- Ashraf MI, Ong SK, Mujawar S, et al. A side-effect free method for identifying cancer drug targets. Sci Rep 2018;8:6669. [Crossref] [PubMed]
- Shammas MA, Shmookler Reis RJ, Koley H, et al. Dysfunctional homologous recombination mediates genomic instability and progression in myeloma. Blood 2009;113:2290-7. [Crossref] [PubMed]
- Pal J, Bertheau R, Buon L, et al. Genomic evolution in Barrett's adenocarcinoma cells: critical roles of elevated hsRAD51, homologous recombination and Alu sequences in the genome. Oncogene 2011;30:3585-98. [Crossref] [PubMed]
- Lu R, Pal J, Buon L, et al. Targeting homologous recombination and telomerase in Barrett’s adenocarcinoma: impact on telomere maintenance, genomic instability and tumor growth. Oncogene 2014;33:1495-505. [Crossref] [PubMed]
- Boysen G, Barbieri CE, Prandi D, et al. SPOP mutation leads to genomic instability in prostate cancer. Elife 2015;4:e09207. [Crossref] [PubMed]
- Faltas BM, Prandi D, Tagawa ST, et al. Clonal evolution of chemotherapy-resistant urothelial carcinoma. Nat Genet 2016;48:1490-99. [Crossref] [PubMed]
- Chen B, Butte AJ. Leveraging big data to transform target selection and drug discovery. Clin Pharmacol Ther 2016;99:285-97. [Crossref] [PubMed]
- Wang RS, Maron BA, Loscalzo J. Systems medicine: evolution of systems biology from bench to bedside. Wiley Interdiscip Rev Syst Biol Med 2015;7:141-61. [Crossref] [PubMed]
- Lahiri C. Disease complexity-A bird’s eye view. J Infect Dis Ther 2015;3:53.
- Carels N, Spinassé LB, Tilli TM, et al. Toward precision medicine of breast cancer. Theor Biol Med Model 2016;13:7. [Crossref] [PubMed]
- Efferth T, Saeed ME, Mirghani E, et al. Integration of phytochemicals and phytotherapy into cancer precision medicine. Oncotarget 2017;8:50284-304. [Crossref] [PubMed]
- Hao DC, Xiao PG. Network pharmacology: a Rosetta Stone for Traditional Chinese Medicine 2014;75:299-312.
- Finkelstein J, Friedman C, Hripcsak G, et al. Potential utility of precision medicine for older adults with polypharmacy: a case series study. Pharmgenomics Pers Med 2016;9:31-45. [Crossref] [PubMed]
- Boland MR, Jacunski A, Lorberbaum T, et al. Systems biology approaches for identifying adverse drug reactions and elucidating their underlying biological mechanisms. Wiley Interdiscip Rev Syst Biol Med 2016;8:104-22. [Crossref] [PubMed]
- Coyle KM, Boudreau JE, Marcato P. Genetic mutations and epigenetic modifications: driving cancer and informing precision medicine. Biomed Res Int 2017;2017:9620870. [Crossref] [PubMed]
- Suarez-Kurtz G. Pharmacogenetic testing in oncology: a Brazilian perspective. Clinics (Sao Paulo) 2018;73:e565s. [Crossref] [PubMed]
- Relling MV, Klein TE. CPIC: clinical pharmacogenetics implementation consortium of the pharmacogenomics research network. Clin Pharmacol Ther 2011;89:464-7. [Crossref] [PubMed]
- Klein TE, Chang JT, Cho MK, et al. Integrating genotype and phenotype information: an overview of the PharmGKB project. Pharmacogenomics J 2001;1:167-70. [Crossref] [PubMed]
- Xu R, Wang Q. An iterative searching and ranking algorithm for prioritising pharmacogenomics genes. Int J Comput Biol Drug Des 2013;6:18-31. [Crossref] [PubMed]
- Garten Y, Coulet A, Altman RB. Recent progress in automatically extracting information from the pharmacogenomic literature. Pharmacogenomics 2010;11:1467-89. [Crossref] [PubMed]
- Chang JT, Altman RB. Extracting and characterizing gene-drug relationships from the literature. Pharmacogenetics 2004;14:577-86. [Crossref] [PubMed]
- Garten Y, Altman RB. Pharmspresso: a text mining tool for extraction of pharmacogenomic concepts and relationships from full text. BMC bioinformatics 2009;10:S6. [Crossref] [PubMed]
- Theobald M, Shah N, Shrager J. Extraction of conditional probabilities of the relationships between drugs, diseases, and genes from PubMed guided by relationships in PharmGKB. Summit Transl Bioinform 2009;2009:124-8. [PubMed]
- Ahlers CB, Fiszman M, Demner-Fushman D, et al. Extracting semantic predications from Medline citations for pharmacogenomics. Pac Symp Biocomput 2007;2007:209-20. [PubMed]
- Coulet A, Shah NH, Garten Y, et al. Using text to build semantic networks for pharmacogenomics. J Biomed Inform 2010;43:1009-19. [Crossref] [PubMed]
- Xu R, Wang Q. A knowledge-driven conditional approach to extract pharmacogenomics specific drug–gene relationships from free text. J Biomed Inform 2012;45:827-34. [Crossref] [PubMed]
- Hansen NT, Brunak S, Altman RB. Generating genome‐scale candidate gene lists for pharmacogenomics. Clin Pharmacol Ther 2009;86:183-9. [Crossref] [PubMed]
- Wu Y, Liu M, Zheng WJ, et al. Ranking gene-drug relationships in biomedical literature using latent dirichlet allocation. Pac Symp Biocomput 2012;2012:422-33. [PubMed]
- Xu R, Wang Q. A semi-supervised approach to extract pharmacogenomics-specific drug–gene pairs from biomedical literature for personalized medicine. J Biomed Inform 2013;46:585-93. [Crossref] [PubMed]
- Prandi D, Baca SC, Romanel A, et al. Unraveling the clonal hierarchy of somatic genomic aberrations. Genome Biol 2014;15:439. [Crossref] [PubMed]
- Van Loo P, Nordgard SH, Lingjærde OC, et al. Allele-specific copy number analysis of tumors. Proc Natl Acad Sci U S A 2010;107:16910-5. [Crossref] [PubMed]
- Greenman CD, Bignell G, Butler A, et al. PICNIC: an algorithm to predict absolute allelic copy number variation with microarray cancer data. Biostatistics 2010;11:164-75. [Crossref] [PubMed]
- Yau C, Mouradov D, Jorissen RN, et al. A statistical approach for detecting genomic aberrations in heterogeneous tumor samples from single nucleotide polymorphism genotyping data. Genome Biol 2010;11:R92. [Crossref] [PubMed]
- Li A, Liu Z, Lezon-Geyda K, et al. GPHMM: an integrated hidden Markov model for identification of copy number alteration and loss of heterozygosity in complex tumor samples using whole genome SNP arrays. Nucleic Acids Res 2011;39:4928-41. [Crossref] [PubMed]
- Imielinski M, Berger AH, Hammerman PS, et al. Mapping the hallmarks of lung adenocarcinoma with massively parallel sequencing. Cell 2012;150:1107-20. [Crossref] [PubMed]
- Berger MF, Hodis E, Heffernan TP, et al. Melanoma genome sequencing reveals frequent PREX2 mutations. Nature 2012;485:502-6. [Crossref] [PubMed]
- Baca SC, Prandi D, Lawrence MS, et al. Punctuated evolution of prostate cancer genomes. Cell 2013;153:666-77. [Crossref] [PubMed]
- Henderson D, Ogilvie LA, Hoyle N, et al. Personalized medicine approaches for colon cancer driven by genomics and systems biology: OncoTrack. Biotechnol J 2014;9:1104-14. [Crossref] [PubMed]
- Weitzel KW, Alexander M, Bernhardt BA, et al. The IGNITE network: a model for genomic medicine implementation and research. BMC Med Genomics 2016;9:1. [Crossref] [PubMed]
- Simmons M, Singhal A, Lu Z. Text mining for precision medicine: bringing structure to EHRs and biomedical literature to understand genes and health. Adv Exp Med Biol 2016;939:139-66. [Crossref] [PubMed]
- Bhuvaneshwar K, Belouali A, Singh V, et al. G-DOC Plus - an integrative bioinformatics platform for precision medicine. BMC Bioinformatics 2016;17:193. [Crossref] [PubMed]
- Kosarek N, Ho ES. IDICAP: a novel tool for integrating drug intervention based on cancer panel. J Pers Med 2016;6:19. [Crossref] [PubMed]
- Law V, Knox C, Djoumbou Y, et al. DrugBank 4.0: shedding new light on drug metabolism. Nucleic Acids Res 2014;42:D1091-7. [Crossref] [PubMed]
- Cotto KC, Wagner AH, Feng YY, et al. DGIdb 3.0: a redesign and expansion of the drug–gene interaction database. Nucleic Acids Res 2018;46:D1068-73. [Crossref] [PubMed]
- Vitali F, Cohen LD, Demartini A, et al. A network-based data integration approach to support drug repurposing and multi-target therapies in triple negative breast cancer. PLoS One 2016;11:e0162407. [Crossref] [PubMed]
- Wathieu H, Issa NT, Fernandez AI, et al. Differential prioritization of therapies to subtypes of triple negative breast cancer using a systems medicine method. Oncotarget 2017;8:92926-42. [Crossref] [PubMed]
- Tran HJ, Speyer G, Kiefer J, et al. Contextualization of drug-mediator relations using evidence networks. BMC Bioinformatics 2017;18:252. [Crossref] [PubMed]
- Hao T, Wang Q, Zhao L, et al. Analyzing of molecular networks for human diseases and drug discovery. Curr Top Med Chem 2018;18:1007-14. [Crossref] [PubMed]
- Szklarczyk D, Franceschini A, Wyder S, et al. STRING v10: protein–protein interaction networks, integrated over the tree of life. Nucleic Acids Res 2015;43:D447-52. [Crossref] [PubMed]
- Kuhn M, Szklarczyk D, Franceschini A, et al. STITCH 3: zooming in on protein–chemical interactions. Nucleic Acids Res 2012;40:D876-80. [Crossref] [PubMed]
- Thurnherr T, Singer F, Stekhoven DJ, et al. Genomic variant annotation workflow for clinical applications. F1000Res 2016;5:1963. [Crossref] [PubMed]
- Mock A, Murphy S, Morris J, et al. CVE: an R package for interactive variant prioritisation in precision oncology. BMC Med Genomics 2017;10:37. [Crossref] [PubMed]
- Ramos AH, Lichtenstein L, Gupta M, et al. Oncotator: cancer variant annotation tool. Hum Mutat 2015;36:E2423-9. [Crossref] [PubMed]
- Cichonska A, Pahikkala T, Szedmak S, et al. Learning with multiple pairwise kernels for drug bioactivity prediction. Bioinformatics 2018;34:i509-18. [Crossref] [PubMed]
Cite this article as: Lahiri C, Pawar S, Mishra R. Precision medicine and future of cancer treatment. Precis Cancer Med 2019;2:33.