Data sharing in the era of precision medicine: a scientometric analysis
Introduction
Precision medicine aims to improve patient outcomes by using the somatic or germline genetic changes in a patient to determine the most effective treatments. The oncology field was considered a precursor in implementing precision medicine in the routine patient care (1). The idea was not new but recent discoveries in computing, biology and bioinformatics helped speed up the pace of this area of research (2). Cost and throughput limitations were the main limitations of DNA sequencing and most of the studies were designed to target a panel of genes of interest or all coding genes (whole exome sequencing) and more recently RNA sequencing. Since 2005, the DNA sequencer companies have made tremendous discoveries and a second-generation sequencing (next generation sequencing) has emerged allowing to conduct deep analysis of complete cancer genomes or exomes (3). As the growth of DNA sequencing increased over the past two decades, the cost per genome dropped from 100 millions dollars in 2001 to reach 1.000 dollars today (4). As the cost of NGS decreased, there would be large amount of data to analyze (5,6). This milestone has changed healthcare models and precision medicine has entered a new era (7,8).
Many countries over the globe launched national precision medicine initiatives in oncology and large amount of clinical and genomic data were collected. Tumor heterogeneity (9) and the accumulation of rare and of unknown significance genomic alterations implied to study hundreds of individuals to identify clinically relevant genomic drivers for treating the disease (10). For rare tumor types, it was crucial to work on an international scale to assemble significant cohorts of patients with the same characteristics. Better the scale was, better our understanding of a disease is or would be. In this context, data sharing appeared as a precondition of the success of precision medicine.
Several countries or funding agencies promoting genomic and clinical data sharing faced challenges at multiple levels (11). Sharing data was governed by both legal and implicit obligations to protect the patient confidentiality and privacy as well as ethical and social issues (12). From the beginning, the bioinformatics community took care in the “FAIR” principle (Findable, Accessible, Interoperable, and Reusable), which led to successful genomic data exchange between the data providers and basic researchers (13). However, sharing genomic along with clinical data was found more difficult in the clinical research community. One reason may be that sharing clinical data required a framework that preserves data quality and patient privacy, and the “FAIR” principles appeared difficult to implement within specific or across health care systems or hospitals. Beyond these technical issues, it took a substantial amount of time, effort and investment to collect the clinical data and in return few organizations wished to share data before conducting their analyses and disseminate their findings (14). In this context, despite the fact that many organizations produced public repositories, data were not shared to a level needed to revolutionize precision medicine.
In this paper, we seek to provide a picture of the current stage of data sharing in precision medicine with a focus on oncology. In order to reach this goal, we performed a scientometric analysis to analyze and measure the science produced from a quantitative perspective. Based on indicators that express scientific activity, this analysis might be useful for guiding subsequent research efforts and help this field move forward.
Methods
Data source
Several publications were analyzed to identify the main databases and tools used in a scientometric analysis (15-17). We used the Clarivate Analytics’s Web of Science (WoS) database, available at http://www.isiknowledge.com. It was chosen because it is one of the world’s premier scientific citation search, discovery, and analytical information platform. It contains tens of millions of bibliographic records covering a broad array of scientific domains.
Search strategies
For choosing the best keywords, we did an inventory of the most commonly encountered terms and tested many string combinations. The most relevant results were obtained using a combined formula: “TI=(data sharing* OR data share*) NOT TI=(SDM* OR shared decision making) AND TS=(precision medicine* OR cancer* OR oncolog* OR health*)”. The “*” is a wildcard that can take any value and TI and TS mean respectively Title and Topic. Our search covered all years between 1900 and 2019 and was performed on August 6, 2019. We retrieved all types of documents including original articles, conference proceedings, review articles, letters, and meeting abstracts.
Data analysis
Specific metrics such as the journal, research area, author, publication year, document type, funding organization and geographical localization were extracted by WoS and analyzed using the Analyze Results function. Impact metrics were also available such as the H-index and the number of citations per year thanks to the Citation report function. As a reminder, a researcher’s H-index means that he/she has at most H papers that were cited at least H times. For instance, an h-index of 10 means there are 10 publications that have 10 citations or more (18,19).
Articles and citations were exported as plain text from WoS to be used by third-party softwares. The VOSviewer (v.1.6.7) (20) was used for analyzing and visualizing bibliometric networks in this paper. The tools are freely available and can take WoS output files as input. VOSviewer has a friendly user interface and performs co-authorship, keyword co-occurence and co-citation map easily.
Results
Total number of publications
A total of 672 documents met the selection criteria during 1990–2019. The most frequent document type was article [400], accounting for 59% of total publications. Proceedings papers were at the second position [136], with a proportion of 20%. Other document types included editorial material [59], review [39], meeting abstract [35], letter [8], news item [8], book chapter [1], data paper [1], and early access [1].
Annual publication and citation number
Figure 1 shows the annual trends of publications and citations. Since the first article was published in 1992, data sharing in oncology obtained very slow increase in the following 10 years. The year 2005 was a turning point with 8 publications and the trend took another turn upward to reach 86–113 publications in the years 2016–2018.
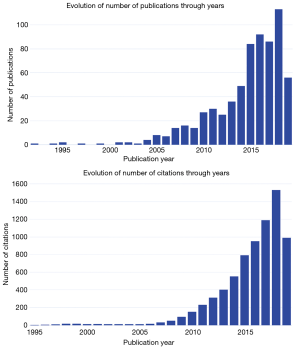
Funding agencies involved
The acknowledgment section of a publication has been indexed since 2008 in WoS (21), explaining the low number of publications illustrated in Table 1. Many funding agencies devoted to promoting data sharing and the major contributors were the United States (US) National Institutes of Health (NIH), the European Commission, and the Wellcome Trust from the United Kingdom.
Table 1
Funding agency | Country/region | Number of publications |
---|---|---|
National Institutes of Health (NIH) | United States | 33 |
European Commission | Europe | 26 |
Wellcome Trust | United Kingdom | 23 |
Medical Research Council (MRC) | United Kingdom | 18 |
National Human Genome Research Institute (NHGRI) | United States | 14 |
National Natural Science Foundation of China (NSFC) | China | 14 |
National Institute on Aging (NIA) | United States | 13 |
German Federal Ministry of Education and Research (BMBF) | Germany | 11 |
Genome Canada | Canada | 6 |
Genome Quebec | Canada | 6 |
Centers for Disease Control and Prevention (CDC) | United States | 6 |
Canadian Institutes of Health Research (CIHR) | Canada | 6 |
Economic and Social Research Council (ESRC) | United Kingdom | 6 |
National Institute for Health Research (NIHR) | United States | 6 |
Agency for Healthcare Research and Quality (AHRQ) | United States | 5 |
Government of Canada | Canada | 4 |
Engineering and Physical Sciences Research Council (EPSRC) | United Kingdom | 4 |
In order to promote data sharing in oncology, many funding agencies required their funded projects to release research data to the scientific community. For each of them, this table displays the number of publications that mentioned his name in the funding acknowledgment section.
Citation and H-index analysis
The WoS Citation report function counted the number of times an article was cited by other works to measure the impact of a publication or author. The number of citations is a useful metric to reflect the quality of a paper. The H-index is another metric to quantify an individual’s scientific research output (see Methods section). According to the analysis of the data from WoS, all publications were cited 7.429 times with an average citation per publication of 11.06. The H-index of all articles was 42.
The USA ranked first with the highest H-index of 32. Germany, Australia and China had almost the same number of publications (43, 44, and 47 respectively), while their citation frequency and H-index were not as comparable, underlying the relevance to include all three parameters in the analysis (Figure 2).
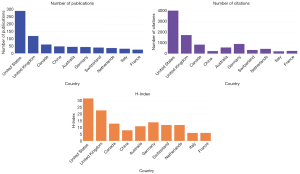
Geographical distribution and author profiles
Due to multiple author affiliations, a total of 903 references were extracted by WoS to study inter-country collaborations on co-authored papers. Contributors included 25 countries. The US, United Kingdom (UK) and Canada were the main contributors with 467 articles (69.49%). The US, with 289 articles (43%) articles, was the most active country and among European countries, the UK, with 118 (17.56%) articles ranked first, followed by Germany. Among Asian countries, China, with 47 (6.99%) articles ranked first (Table 2).
Table 2
Name of country | Number of publications (% of 672) | Top-ranked authors* |
---|---|---|
United States | 289 (43.00) | Ohno-Machado, Lucila |
United Kingdom | 118 (17.56) | Parker, Michael |
Canada | 60 (8.92) | Knoppers, Bartha M. |
China | 47 (6.99) | |
Australia | 44 (6.54) | Chalmers, Donald |
Germany | 43 (6.39) | |
Switzerland | 39 (5.80) | |
Netherlands | 36 (5.35) | |
Italy | 31 (4.61) | |
France | 25 (3.72) | |
Scotland | 23 (3.42) | |
Belgium | 20 (2.97) | |
Spain | 17 (2.53) | |
Japan | 14 (2.08) | |
South Korea | 14 (2.08) | |
India | 12 (1.78) | |
Sweden | 12 (1.78) | |
Thailand | 11 (1.63) | |
Denmark | 8 (1.19) | |
South Africa | 8 (1.19) | |
Northern Ireland | 7 (1.04) | Lawler, Mark |
Saudi Arabia | 7 (1.04) | |
Austria | 6 (0.89) | |
Greece | 6 (0.89) | Verropoulou, Georgia |
Ireland | 6 (0.89) |
Data sharing in oncology around the world was very disparate and scientists from countries that were the most economically developed increased their efforts to share data. This table summarizes the top-rank countries along with the number of publications and frequencies. For each of them, the name of the author that published the most is displayed.
WoS provided the top-ranked authors that published in the field. We investigated the main expertise of the top three of them. Parker Michael, from the University of Oxford, with 13 publications (1.93%) had the highest number of publications. This professor of bioethics is interested in the clinical use of genetics. Knoppers Bartha, from McGill University, with 12 publications (1.78%) is an expert on the ethical aspects of genetics and genomics. Ohno-Machado Lucila, from the University of San Diego, CA, is interested in biomedical informatics and was involved in 8 publications (1.19%).
The VOSViewer visualization software allowed us to build a network of co-authorship by country (Figure 3A). The United States was the main contributor. In Europe, the United Kingdom and Germany were the top-ranked countries. China and Japan were the main contributors in Asia.
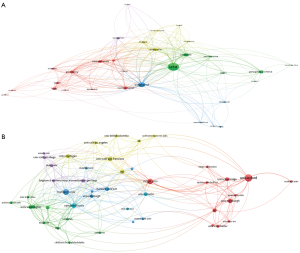
Institutions contribution
We then focused our analysis on institutions involved in the data sharing initiatives. Using WoS results we found that 25 institutions contributed in publishing papers in the field. Seventeen of them were from the US, two were from Canada, and five are from the UK. The vast majority were universities: Oxford University with 32 publications (4.7%), Harvard University with 23 publications (3.42%), and McGill with 21 articles (3.12%) were the top three universities (Table 3).
Table 3
Organizations | Country | Number of publications (% of 672) |
---|---|---|
University of Oxford | United Kingdom | 32 (4.70) |
University of Harvard | United States | 23 (3.42) |
McGill University | Canada | 21 (3.12) |
University of Vanderbilt | United States | 17 (2.53) |
University of California, San Francisco (UCSF) | United States | 15 (2.23) |
University of Edinburgh | United Kingdom | 15 (2.23) |
Harvard Medical School | United States | 13 (1.93) |
National Cancer Institute (NCI) | United States | 13 (1.93) |
University College London (UCL) | United Kingdom | 13 (1.93) |
University of Michigan | United States | 13 (1.93) |
University of Cambridge | United Kingdom | 12 (1.78) |
University of Toronto | Canada | 12 (1.78) |
University of Washington | United States | 12 (1.78) |
Baylor College of Medicine | United States | 11 (1.63) |
Massachusetts General Hospital | United States | 11 (1.63) |
Northwestern University | United States | 11 (1.63) |
Duke University | United States | 10 (1.48) |
University of Pittsburgh | United States | 10 (1.48) |
University of California San Diego (UCSD) | United States | 9 (1.33) |
Brigham and Women’s Hospital | United States | 8 (1.19) |
Chinese Academy of Sciences | China | 8 (1.19) |
The University of British Columbia | United States | 8 (1.19) |
The University of California, Los Angeles (UCLA) | United States | 8 (1.19) |
The University of Manchester | United Kingdom | 8 (1.19) |
The University of Arizona | United States | 7 (1.04) |
The principal organizations involved in this field were from prestigious universities and respected research institutes. This table displays the most-cited organizations and, for each of them, the number of publications and the corresponding frequency.
The VOSViewer visualization software allowed us to build a network of co-authorship by institution (Figure 3B). Over the 1,117 registered organizations, a threshold of six publications was met by 48 institutions. A co-authorship link strength was calculated between each institution enabling them to be classified in different clusters. The size of the circle of a node was proportional to the number of articles. The color of a node indicated the cluster to which it belonged to and the distance between two nodes indicated their relatedness. Of note, the closer two institutions were located to each other, the stronger their collaboration was. The network highlighted that collaborative efforts in data sharing were mostly the result of collaborations between geographically close universities. Oxford collaborated mainly with Edinburgh, Cambridge, Manchester, King’s College London and University College London, while Harvard University collaborated mainly with Harvard Medical School, Stanford University, and University of Pittsburgh.
Research areas
Every journal and book covered by the WoS Core Collection was assigned to at least one research area. A total of 932 topics were identified, reflecting that a wide variety of journals published in this field. Computer science, with 164 articles (24.4%), health care sciences services with 120 articles (17.85%) and medical informatics with 85 articles (12.64%) were the top ranked research areas. A total of 277 (41.22%) publications were in the field of computer knowledge (computer science, medical informatics, telecommunications, mathematical and computational biology), underlying the fact that data sharing faced major technical challenges (Table 4).
Table 4
Research areas | Number of publications (% of 672) |
---|---|
Computer science | 164 (24.40) |
Health care sciences & services | 120 (17.85) |
Medical informatics | 85 (12.64) |
The environmental and occupational health | 72 (10.71) |
Engineering | 57 (8.48) |
General internal medicine | 52 (7.73) |
Genetics heredity | 50 (7.44) |
Social science other topics | 32 (4.76) |
Oncology | 31 (4.61) |
Telecommunications | 28 (4.16) |
Science technology other topics | 27 (4.01) |
Library and information science | 26 (3.86) |
Biochemistry and molecular biology | 25 (3.72) |
Medical ethics | 24 (3.57) |
Mathematical and computational biology | 17 (2.53) |
Business economics | 14 (2.08) |
Neurosciences neurobiology | 14 (2.08) |
Research in experimental medicine | 14 (2.08) |
Biomedical social science | 13 (1.93) |
Biotechnology applied microbiology | 13 (1.93) |
Government laws | 13 (1.93) |
Cell biology | 11 (1.63) |
Cardiovascular system cardiology | 10 (1.48) |
Geriatrics & gerontology | 10 (1.48) |
Mathematics | 10 (1.48) |
Journals covered by WoS core collection are assigned to at least one research area. This table summarizes the most important categories involved in the dissemination of the research data in precision medicine. For each of them, we display the number of publications and the corresponding frequency.
Journal co-citation analysis
A journal co-citation analysis compiled the number of times two journal titles were jointly cited in later publications. It is an efficient way to study the structure and the characteristics of a subject (22). VOSViewer was used to plot the journal co-citation network. Figure 4 shows the clustering result of this analysis. Among a total of 7,549 journals, only 109 journals met the threshold of a minimum of 20 citations. The journal co-citation network identified five clusters. Each node had a color indicating the cluster to which it belonged. The distance between two nodes indicated the citation frequency between two journals. The smaller the distance between two nodes was, the higher the citation frequency was. The red and purple clusters contained high impact general medical journals such as Journal of the American Medical Association, New England Journal of Medicine and The Lancet. The green and blue clusters included high impact journals such as Nature and Science that are focused on science. Finally, the yellow cluster included medical informatics journals such as The Journal of the American Medical Informatics Association, Lecture Notes in Computer Science and The Journal of Medical Internet Research.
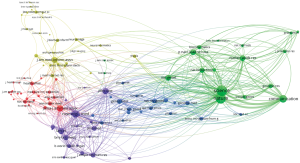
Term co-occurrences map
VOSViewer was used to create a map based on co-occurrences of terms in titles and abstracts of publications. A total of 14,610 terms were retrieved using an automatic term identification technique and 433 terms met the 10 minimum number of occurrences threshold. For each of the term, a relevance score was calculated and we selected all of them. The keyword “data” appeared first with 1,857 occurrences. For the sake of clarity, we manually selected the terms related to “data”. For instance, we kept the terms “policy”, “system”, “privacy”, “sharing”, and “access”, and excluded “participant”, “paper”, “country”, “time”, “article” etc. In total, 79 terms were chosen and a map was built using the association strength normalization (Figure 5A). The algorithm produced five clusters (yellow, red, blue, purple and green). The size of the labels and nodes represented the weights of the nodes. The distance between two nodes represented the strength of the relation between them and finally the thicker a line was, the more co-occurrence they had. The keyword “data sharing” had the highest frequency of 441 occurrences followed by “system” [360], “sharing” [265], “model” [265], “use” [208], “policy” [190], “privacy” [185], and “access” [147]. The link strength between two nodes was used as a quantitative index to depict the relationship between two nodes.
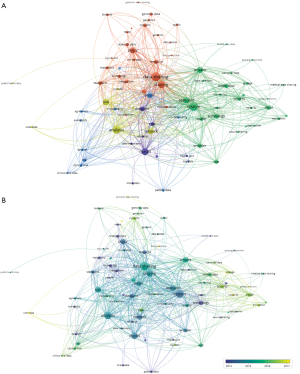
The relationships between “data sharing” and “system”, “cloud”, “technology”, “cloud”, “network” and “database” reflected the importance of computing science. The relationship between “data sharing” and “privacy”, “policy”, and “consent” showed the importance of issues related to patient’s privacy and confidentiality.
The overlay visualization showed the emergence of the keywords over time (Figure 5B). This map showed that the keywords such as “cloud”, “encryption”, “security”, “integrity”, “interoperability” were relatively new to this area of research. Their association in the same cluster indicated that cloud computing was associated with new security challenges.
Discussion
The goal of this work was to produce an update of the current stage of data sharing in precision medicine with a focus on oncology. This was done using a scientometric approach to provide both a qualitative and quantitative perspective to the scientific production in this field. Our work highlighted a number of important observations. This area of research is relatively new, with a rapid growth of publications and citations from 2005, and an unequal quantitative production of scientific literature across countries and institutions. Interdisciplinarity is a hallmark of this field and appears necessary to overcome major technical challenges that we are documenting through term occurrences.
The main reasons for the recent emergence of data sharing in the context of precision medicine in oncology are: (I) the digital revolution in the sharing of scientific information across the internet and (II) the emergence of NGS that allowed a high-throughput and less expensive sequencing. Within the frame of national and international initiatives, mostly funded by United States and European agencies, the importance of making cancer genomics data publicly available to accelerate the progress of research was acknowledged and guidelines by various sponsored projects. After the Sanger Institute delivered one-third of the successful Human Genome Project in 2003 (23), the Wellcome Trust announced in 2005 a five-year investment of £340 million to the Sanger Institute. Cancer projects such as the Cancer Genome Project focused on breast, lung and kidney and researchers were strongly encouraged to release large-scale datasets (24). In the US, the National Institutes of Health (NIH) required investigators to share their data as part of their funding applications (25). In 2005, the NIH officially launched The Cancer Genome Atlas (TCGA) to accelerate the understanding of the molecular basis of cancer with a three-year investment of $100 million (26). This project was then extended in 2010 to increase in scale and to extend over wider diseases (27). During this period, the International Cancer Genome Consortium (ICGC) was launched in 2008 with an active policy to allow data sharing (28). The European Commission funded projects involved in cancer research through the sixth (FP6 2002–2006) and seventh (FP7 2007–2013) framework programs. FP7 dedicated €1.1 billion to cancer research with a strong focus on cross-border collaborations amongst cancer centers. For example, the Eurocan Platform project had a €12 million budget to develop a shared platform across 28 leading cancer institutes (29) to foster sharing of data and samples, such as the Organisation of European Cancer Institute (OECI)-Tubafrost Central Database and the European Organisation for Research and Treatment of Cancer (EORTC) that offers a trial data sharing facility. As a consequence of this evolution, a large amount of data is now available around the world that can be retrieved through various ways (http://www.cbioportal.org/; https://portal.gdc.cancer.gov/). Of note, publicly available genomic and clinical data involves patients outside clinical trials in the vast majority of the cases; hence, clinical data is often limited.
Data sharing in precision medicine is a multidisciplinary effort involving experts in various fields. The term co-occurrences map reflects the main parameters, challenges and obstacles that govern data sharing. Computer scientists face considerable challenges related to data storage, safety, interoperability, and data access from multiple repositories or clouds. Experts in ethics focus of how research could impact the privacy and confidentiality of patients. In addition to these technical and social issues, collecting and sharing data is expensive. While funding agencies exhorted researchers to share resources and data, the progress has been slowed down by practical and cultural barriers within the context of a highly competitive environment (30). Finally, our analysis shows that the involvement of clinicians in the context of clinical trials is very recent [2015] but is critical to allow high-throughput data to be integrated with high quality clinical data. Pharmaceutical companies have recently started to implement policies to promote data sharing (31,32). However, a recent study has shown that as few as 15% clinical trials were available for data sharing 2 years after publication of primary results of the trial, underlying that most industry sponsors have no data sharing policy (33).
The distribution of the quantitative production of scientific literature across countries and more precisely across institutions showed an important difference. Northern America and Western Europe accounted in about 80% of the total production with key contributors such as the US and the UK. Data protection around the world is heterogeneous and many funding agencies and countries have enforced regulations around data privacy and protection (34,35). This may explain why data sharing involves preferentially academic institutions from the same country and/or that are close to each other. Efforts such as multi-phase, multi-year, international project form the American Association for Cancer Research (AACR) Project Genomics Evidence Neoplasia Information Exchange (GENIE) is to be commended (36).
Our work has some limitations. WoS and Scopus are the most widespread databases on different scientific fields that are frequently used for searching in literature. The selection of one or the other depends on the disciplines, document type, and the time period of research cited. Even though Scopus has, in general, a slightly better coverage in biomedical research (37), we selected WoS for the quality of the key metrics used in our review such as a better coverage of funding information (38). (I) A small dataset of publications to conduct a scientometric analysis; and (II) the search string that we extended beyond the precision medicine field that led to “false positives” publications; and (III) the exclusive use of the WoS database not combined with other search engines (i.e., Scopus, Google Scholar) which could lead to different results and conclusions.
To conclude, our work outlines that the emergence of this field is recent, is marked by interdisciplinarity and illustrates bottlenecks. The scientific production related to data sharing in precision medicine is growing worldwide, with marked differences among continents in terms of quantity of the production. Our data may contribute in tacking a picture of the field, which could be of interest for all stakeholders in precision medicine in oncology.
Supplementary
Acknowledgments
We would like to thank all of the participants of the OSIRIS project (GrOupe inter-SIRIC sur le paRtage et l’Intégration des donnéeS clinico-biologiques en cancérologie).
Funding: LYriCAN INCa-DGOS-Inserm_12563; OSIRIS (GrOupe inter-SIRIC sur le paRtage et l’Intégration des donnéeS clinico-biologiques en cancérologie) supported by the Institut National du Cancer (INCa_12600).
Footnote
Conflicts of Interest: All authors have completed the ICMJE uniform disclosure form (available at http://dx.doi.org/10.21037/pcm.2019.09.02). The authors have no conflicts of interest to declare.
Ethical Statement: All authors are accountable for all aspects of the work (if applied, including full data access, integrity of the data and the accuracy of the data analysis). Questions related to the accuracy or integrity of any part of the work are appropriately investigated and resolved. Data extracted from Web of Sciences and used for analysis is provided as supplementary material.
Open Access Statement: This is an Open Access article distributed in accordance with the Creative Commons Attribution-NonCommercial-NoDerivs 4.0 International License (CC BY-NC-ND 4.0), which permits the non-commercial replication and distribution of the article with the strict proviso that no changes or edits are made and the original work is properly cited (including links to both the formal publication through the relevant DOI and the license). See: https://creativecommons.org/licenses/by-nc-nd/4.0/.
References
- Verma M. Personalized Medicine and Cancer. J Pers Med 2012;2:1-14. [Crossref] [PubMed]
- Ravegnini G, Angelini S. Toward Precision Medicine: How Far Is the Goal? Int J Mol Sci 2016;17:245. [Crossref] [PubMed]
- Kchouk M, Gibrat JF, Elloumi M. Generations of Sequencing Technologies: From First to Next Generation. Biology and Medicine 2017;9:3. [Crossref]
- The Cost of Sequencing a Human Genome [Internet]. National Human Genome Research Institute (NHGRI). [cited 2018 Dec 13]. Available online: https://www.genome.gov/27565109/the-cost-of-sequencing-a-human-genome/
- Muir P, Li S, Lou S, et al. The real cost of sequencing: scaling computation to keep pace with data generation. Genome Biol 2016;17:53. [Crossref] [PubMed]
- Stephens ZD, Lee SY, Faghri F, et al. Big Data: Astronomical or Genomical? PLoS Biol 2015;13:e1002195. [Crossref] [PubMed]
- Scollen S, Page A, Wilson J. Health on behalf of the GA for G and. From the Data on Many, Precision Medicine for “One”: The Case for Widespread Genomic Data Sharing. BMH 2017;2:15.
- Blasimme A, Fadda M, Schneider M, et al. Data Sharing For Precision Medicine: Policy Lessons And Future Directions. Health Aff (Millwood) 2018;37:702-9. [Crossref] [PubMed]
- Burrell RA, McGranahan N, Bartek J, et al. The causes and consequences of genetic heterogeneity in cancer evolution. Nature 2013;501:338-45. [Crossref] [PubMed]
- Chin L, Hahn WC, Getz G, et al. Making sense of cancer genomic data. Genes Dev 2011;25:534-55. [Crossref] [PubMed]
- Figueiredo AS. Data Sharing: Convert Challenges into Opportunities. Front Public Health 2017;5:327. [Crossref] [PubMed]
- Minari J, Brothers KB, Morrison M. Tensions in ethics and policy created by National Precision Medicine Programs. Hum Genomics 2018 17;12:22.
- Corpas M, Kovalevskaya NV, McMurray A, et al. A FAIR guide for data providers to maximise sharing of human genomic data. PLOS Computational Biology 2018;14:e1005873. [Crossref] [PubMed]
- Enabling the effective sharing of clinical data : Scientific Data [Internet]. [cited 2018 Dec 13]. Available online: http://blogs.nature.com/scientificdata/2016/05/13/enabling-the-effective-sharing-of-clinical-data/
- Shao H, Yu Q, Bo X, et al. Analysis of oncology research from 2001 to 2010: a scientometric perspective. Oncol Rep 2013;29:1441-52. [Crossref] [PubMed]
- Liao H, Tang M, Luo L, et al. A Bibliometric Analysis and Visualization of Medical Big Data Research. Sustainability 2018;10:166. [Crossref]
- Thonon F, Boulkedid R, Delory T, et al. Measuring the Outcome of Biomedical Research: A Systematic Literature Review. PLoS One 2015;10:e0122239. [Crossref] [PubMed]
- Hirsch JE. An index to quantify an individual’s scientific research output. Proc Natl Acad Sci USA 2005;102:16569-72. [Crossref] [PubMed]
- Bornmann L, Daniel HD. What do we know about the h index? J Am Soc Inf Sci Technol 2007;58:1381-5. [Crossref]
- van Eck NJ, Waltman L. Software survey: VOSviewer, a computer program for bibliometric mapping. Scientometrics 2010;84:523-38. [Crossref] [PubMed]
- Web of Science Core Collection Help [Internet]. [cited 2018 Dec 13]. Available online: https://images.webofknowledge.com/images/help/WOS/hp_full_record.html
- Trujillo CM, Long TM. Document co-citation analysis to enhance transdisciplinary research. Science Advances 2018;4:e1701130. [Crossref] [PubMed]
- Green ED, Watson JD, Collins FS. Human Genome Project: Twenty-five years of big biology. Nature 2015;526:29-31. [Crossref] [PubMed]
- www-core (webteam). Tackling the Basis (and Bases) of Disease [Internet]. [cited 2018 Dec 13]. Available online: https://www.sanger.ac.uk/news/view/2005-12-21-tackling-the-basis-and-bases-of-disease
- NIH Guide: FINAL NIH STATEMENT ON SHARING RESEARCH DATA [Internet]. [cited 2018 Dec 13]. Available online: https://grants.nih.gov/grants/guide/notice-files/NOT-OD-03-032.html
- NIH Launches Comprehensive Effort to Explore Cancer Genomics [Internet]. The Cancer Genome Atlas - National Cancer Institute 2005 [cited 2018 Dec 13]. Available online: https://cancergenome.nih.gov/newsevents/newsannouncements/news_12_13_2005
- Recovery Act Investment Enables TCGA to Map 20 Cancers [Internet]. The Cancer Genome Atlas - National Cancer Institute 2010 [cited 2018 Dec 13]. Available online: https://cancergenome.nih.gov/newsevents/newsannouncements/news_9_30_2009
- International network of cancer genome projects. Nature 2010;464:993-8. [Crossref] [PubMed]
- van de Loo JW, Trzaska D, Berkouk K, et al. Emphasising the European Union’s Commitment to Cancer Research: A Helicopter View of the Seventh Framework Programme for Research and Technological Development. Oncologist 2012;17:e26-32. [Crossref] [PubMed]
- Alter GC, Vardigan M. Addressing Global Data Sharing Challenges. J Empir Res Hum Res Ethics 2015;10:317-23. [Crossref] [PubMed]
- Ayers M, Nebozhyn M, Cristescu R, et al. Molecular Profiling of Cohorts of Tumor Samples to Guide Clinical Development of Pembrolizumab as Monotherapy. Clin Cancer Res 2019;25:1564-73. [Crossref] [PubMed]
- Merck Procedure on Clinical Trial Data Access Final_Updated July_9_2014.pdf [Internet]. [cited 2019 Jan 25]. Available online: https://www.merck.com/clinical-trials/pdf/Merck%20Procedure%20on%20Clinical%20Trial%20Data%20Access%20Final_Updated%20July_9_2014.pdf
- Hopkins AM, Rowland A, Sorich MJ. Data sharing from pharmaceutical industry sponsored clinical studies: audit of data availability. BMC Med 2018;16:165. [Crossref] [PubMed]
- Guttmacher AE, Nabel EG, Collins FS. Why data-sharing policies matter. PNAS 2009;106:16894. [Crossref] [PubMed]
- Sorani MD, Yue JK, Sharma S, et al. Genetic data sharing and privacy. Neuroinformatics 2015;13:1-6. [Crossref] [PubMed]
- Consortium TAPG. AACR Project GENIE: Powering Precision Medicine through an International Consortium. Cancer Discov 2017;7:818-31. [Crossref] [PubMed]
- Mongeon P, Paul-Hus A. The journal coverage of Web of Science and Scopus: a comparative analysis. Scientometrics 2016;106:213-28. [Crossref]
- Kokol P, Vošner HB. Discrepancies among Scopus, Web of Science, and PubMed coverage of funding information in medical journal articles. J Med Libr Assoc 2018;106:81-6. [Crossref] [PubMed]
Cite this article as: Le Texier V, Henda N, Cox S, Rousseau-Tsangaris M, Saintigny P. Data sharing in the era of precision medicine: a scientometric analysis. Precis Cancer Med 2019;2:30.